Breeding with PGR
Background
The collection, description and storage of plant collections (seeds, plant material) is one aspect of plant genetic resources, the other is the utilization of this variation for plant breeding. Genetic resources are important and interesting for plant breeding for the following reasons:
- Source of new disease resistance genes
- Source of new genes for drought and other abiotic stress tolerance
- Source of genes for new traits such as quality traits
- Restoration of traits that were lost during domestication
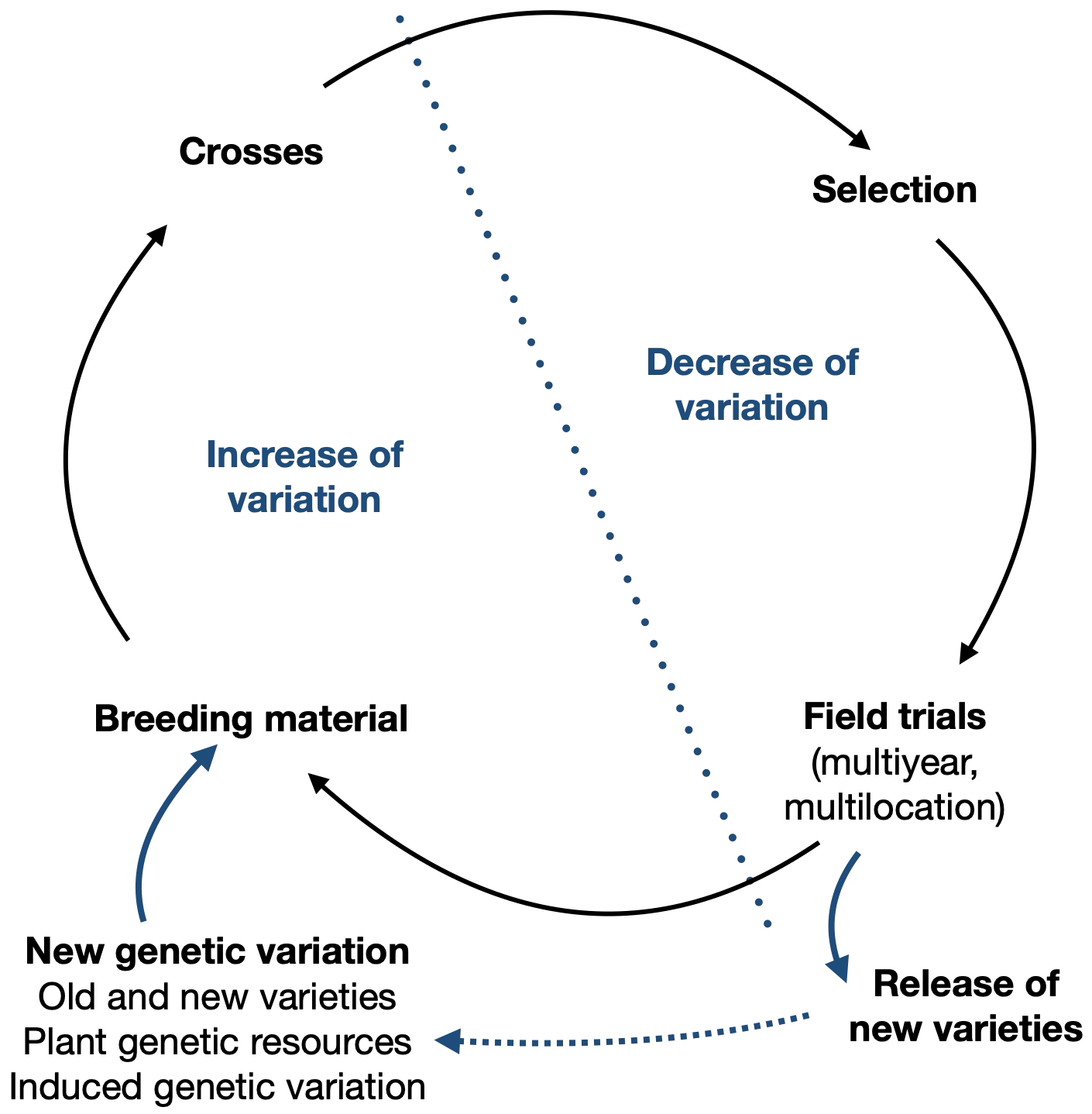
The use of genetic resources is particularly useful in one part of the plant breeding cycle, the creation of novel genetic variation in a breeding population (Figure 1).
In many crops, domestication and modern plant breeding have led to the genetic erosion of variation, hence wide crosses with exotic material are a potentially useful approach to generate new genetic diversity in a crop species. It is also a means to re-introduce useful alleles that were ‘left behind’ in the wild species during domestication (McCouch, 2004). Introgression of exotic alleles provides the chance to generate new combinations of productive genotypes that possibly exceeds the trait values of the parents, a process that is called transgressive segregation. An equivalent to transgressive segregation would be to find those genetic resources that produce higher phenotypic values in elite x resources crosses versus in (frequently genetically more narrow) elite x elite crosses (Figure 2).
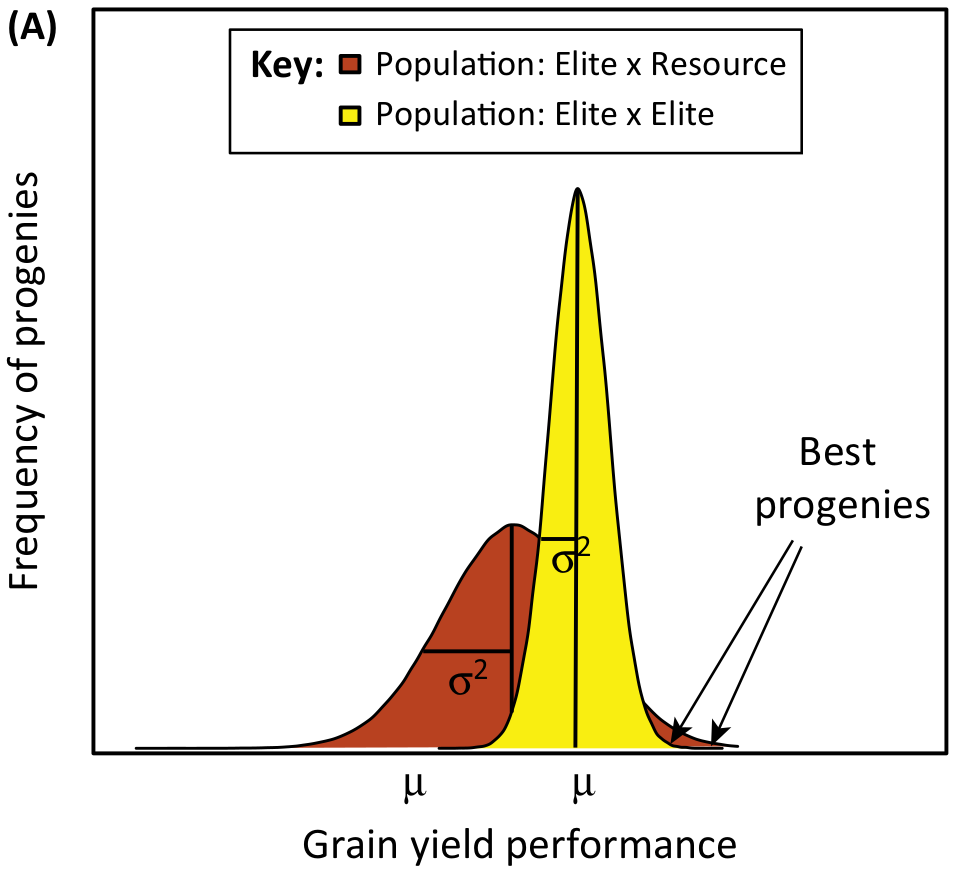
However, the introduction of new genetic variation into a breeding population has a positive and a negative aspect. Not only useful genes, but also disadvantageous genes are introduced into elite breeding populations. The water melon is a good example (Figure 3). Wild watermelon species have dominant genes that cause an extremely bitter taste and a white fruit flesh. Domesticated varieties probably originated through the selection of rare recessive genes causing non-bitterness and a red flesh (Figure 3). Any introduction of favorable genes into the cultivated from the wild watermelon needs to make sure that the disadvantageous traits are not introgressed as well. This goal may present a formidable challenge for a breeder if such disadvantageous traits have a dominant gene action and are genetically linked to recessive genes with positive effects on a phenotypic trait.
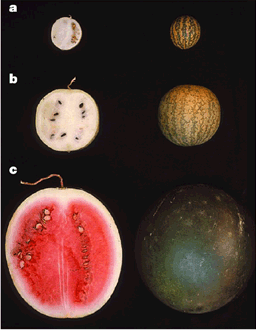
For this reason, breeders are often reluctant to open their gene pools to exotic genetic variation because any breeding progress may be destroyed easily. Instead, a carefully designed pre-breeding schemes are frequently used for the introgression of new genetic variation.
Learning goals
Problems with introgression of exotic diversity
As has already been discussed in the context of the gene pool concept in chapter genetic-diversity.qmd, the introduction of exotic germ plasm has a high risk of failure for several reasons:
- Cross or hybrid incompatibility between wild and crop species.
- Sterility of the F\(_1\) hybrid progeny of parents from different gene pools.
- Infertility of the segregating generations.
- Reduced recombination between the chromosomes of the two species.
- Linkage drag due to tight linkage of genes with a negative effect to the trait of interest.
Figure 4 shows that genetic resources can not be directly used and therefore need to be refined by appropriate backcrossing. In this example, late-flowering Peruvian landraces were crossed into European elite varieties to delay flowering and to increase the accumulation of biomass during the growing season. The breeding goal is to produce maize for bioenergy production. However, the large plants show a high propensity for lodging, and need to be further bred to increase lodging resistance.
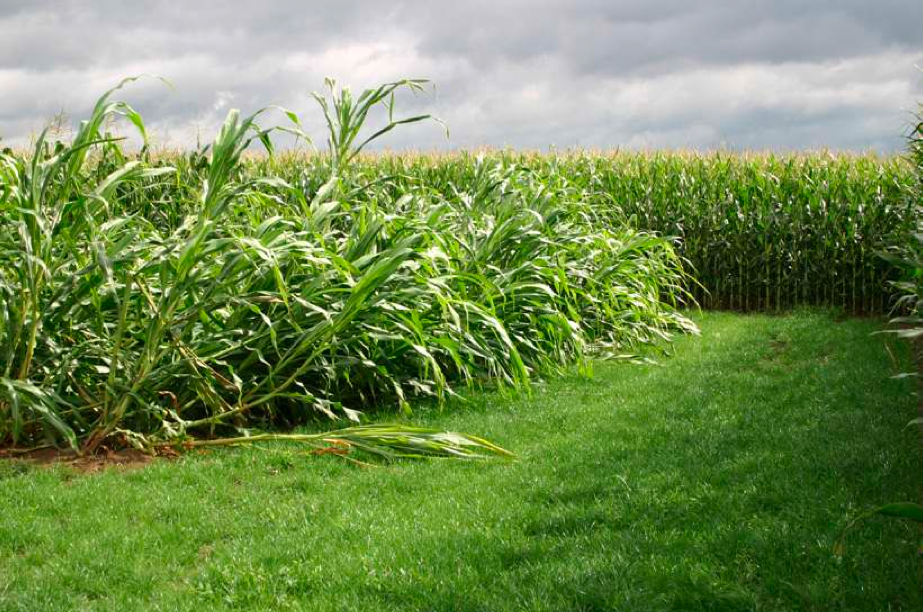
Another important aspect concerns the genetic architecture of introgressed traits. Monogenic (i.e., Mendelian) traits are relatively easy to introgress, but quantitative traits that are controlled by multiple genes (Quantitative trait loci, QTLs) are much more difficult to introgress into elite varieties.
The most important methods for the introgression of PGR into modern varieties are:
- Backcrossing
- Pyramiding
- Genetic engineering
Many genetic resources are available for prebreeding
Several projects screen genetic resources from genebanks and define core collections based on maximized genetic distances that are then used to evaluate their potential per se or in testcrosses with elite tester lines.
Another approach is to create new synthetic crops that are maximized for genetic diversity. For example, new synthetic wheat genotypes have been created that are based on maximized genetic diversity in the D genome of wheat (e.g., WISP, CIMMYT) Rosyara et al. (2019).
A key question is which genetic resources are suited for elite breeding and how they can be efficiently identified and characterized. For this reason, there are genetic resources and before starting a prebreeding program, numerous questions have to be addressed:
- What is the optimal breeding scheme to breed into elite varieties?
- Which elite parents are suitable for introgression?
- How to select the optimal offspring and which traits are most suitable?
- Which approach is most efficient given the available resources?
Considerations for optimizing prebreeding
Several aspects need to be considered to make a prebreeding program successful:
- Size of field trials
- Which traits should be phenotyped at which state of development and at which stage in the breeding program?
- Which breeding approach should be used?
- What is the optimal experimental design for prebreeding?
Since plant breeding is a numbers game (larger experiments are usually better), it is necessary to use experiments with a high statistical power for high genetic variation.
For example, in the projects Zuchtwert and Genebank 2.0 the German genebank at IPK and the State Breeding Institute (LSA) in Hohenheim established 2,000 observation rows at 2 locations, for diseases like rusts, mildew and septoria, as well as heading, plant height and frost tolerance (Schulthess et al., 2022).
Since one of the goals of these trials was hybrid seed production (in a mainly self-fertilizing crop), more than 300 genetic resources were crossed with few elite testers, and a gametozide-based (i.e., chemical) sterilization had to be used because no genetic male-sterility system is currently available for wheat.
For hybrid yield tests, more than 300 of the resulting hybrids between genetic resources and elite testers were tested in yield trials in at least five locations and more than three locations with observation trials. This experiment allowed to identify traits with major QTLs. For disease resistance, special qualities, (e.g., high-molecular weight (HMW) and low-molecular weight (LMW) glutenin), plant height, heading date were identified, and the challenge was to identify new major QTLs behind new resistances and new genes for HMW.
This research showed genetic resources can be correctly screened in the field with accepbudget requirements to identify new major QTLs providing disease resistance and new HMW glutenin traits. Once the candidate QTLs are identified, future steps are to validate these QTLs in elite backgrounds, develop markers and strategies for marker-aided backcrossing and allele mining of these QTLs in all resources.
Challenges in the phenotypic evaluation of plant genetic resources
The evaluation of complex traits such as grain yield in plant genetic resources is challenging for various reasons. As already mentioned in a previous section, strong GxE interactions frequently observed in genetic resources may prevent the reliable measurement of phenotypic traits. One example is shown in Figure 5, where a tendency to lodging under nitrogen fertilization interferes with the analysis of grain yield. As a result, the trait values maybe of too poor quality to reliably identify QTLs because the phenotypic values can not be compared to elite “check” varieties that are used for comparison.
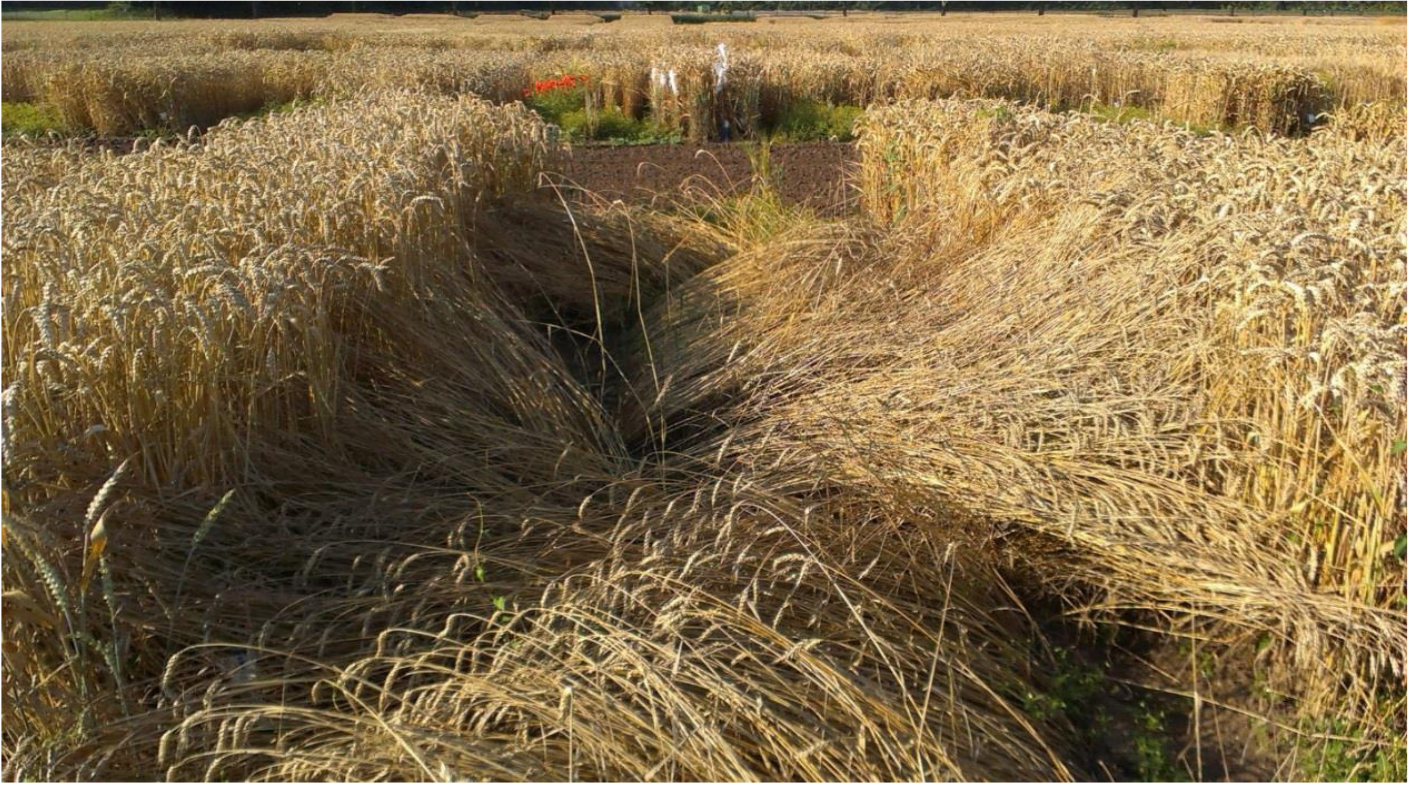
Therefore, genetic resources are frequently not used as varieties themselves, but as parents for crosses to elite lines. In hybrid breeding, the per se performance of parental lines is frequently of great interest because it contributes to the performance of the hybrid. In the case of exotic genetic resources as parents, the per se performance is frequently not of interest, because it is reduced by deleterious variants, and the identification of individual QTLs that can be later introgressed into breeding material is of greater interest (Mayer et al., 2020).
If the identification of useful QTLs is the main interest in the breeding-related evaluation of PGR, the allocation of available resources is an important decision to make. Assume that the available budget allows to evaluate a total of 10,000 new DH lines created from genetic resources as crossing partners for elite varieties. The main question to answer is then:
- Many crosses with few progenies per cross?
- Few crosses with many progenies per cross?
Successful breeders use different strategies, e.g.,
- 50 crosses each with 200 DHs
- 500 crosses each with 20 DHs
Theoretical analyses based on simulations suggest that the parental performance is more important than the number of crosses versus the size of a cross (Bernardo, 2003). Figure 6 shows that both the mean and the standard deviation among progeny of crosses do not change much with the size of a breeding population, which is influenced by the number of crosses and their size, but more by the performance of the parents. In particular, the mean trait value is higher if the top 10% of the parents are selected for the crosses in comparison to the top 25% of parents or random parents from a parental population. These results are robust for different levels of heritability and suggest that a prior evaluation of plant genetic resources is an important component in the utilization for plant breeding.
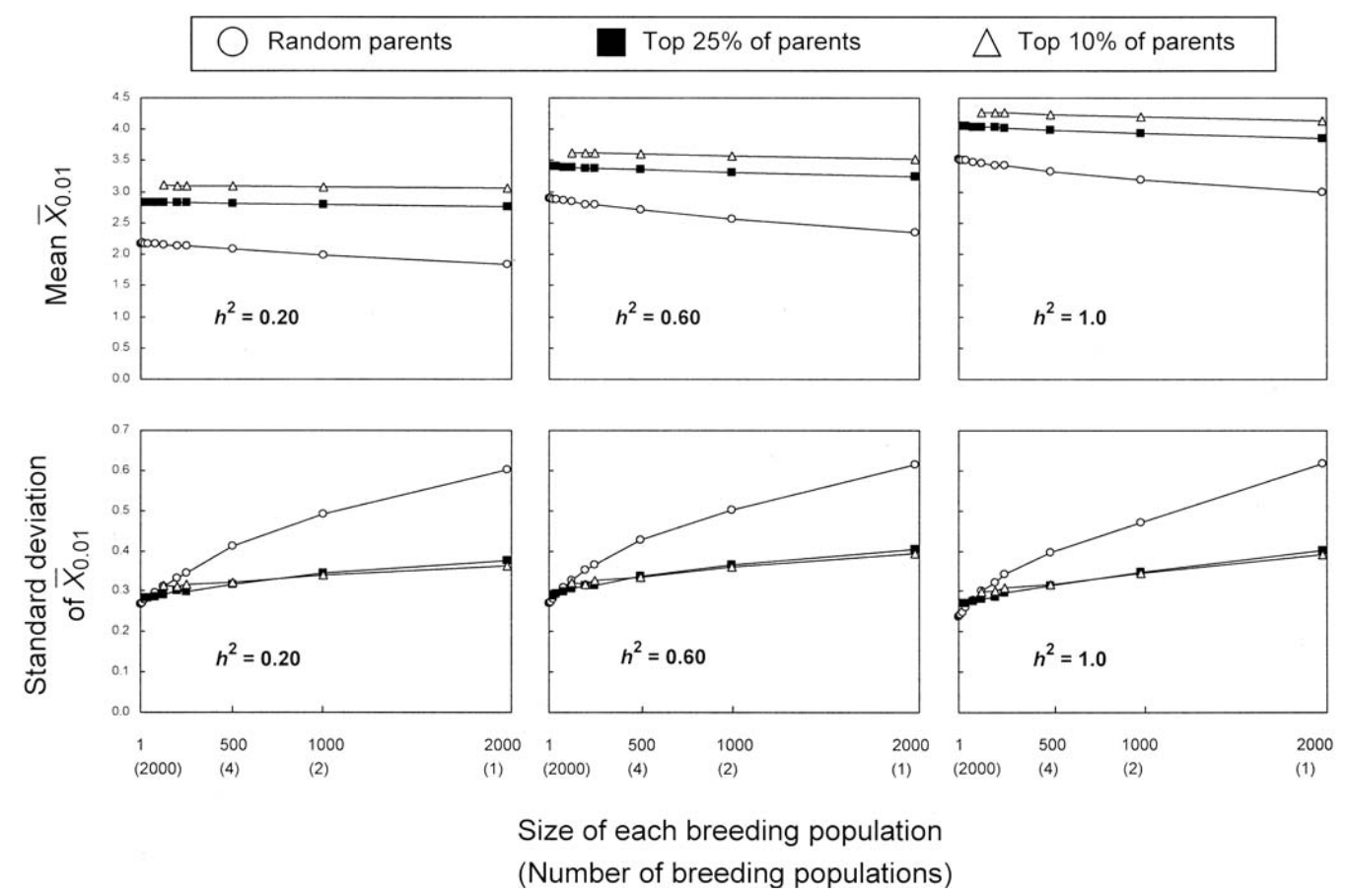
Another consideration relates to the role of the genetic architecture of the phenotypic traits of interest. This is because the probability of discovering an optimal genotype decreases with the number of segregating QTLs, as shown in Table 1.
The more complex the trait, the more progenies are necessary to find the “perfect” genotype, which combines the positive alleles of all QTLs present among the parents.
Loci | \(F_{2}\) (recessive) | \(F_{2}\) (dominant) |
---|---|---|
1 | \(1/4\) | \(3/4\) |
4 | \(1/256\) | \(1/3\) |
8 | \(1/65,563\) | \(1/10\) |
16 | \(1/4,294,967,296\) | \(1/100\) |
40 | \((1/4)^{40}\) | \(1/100,000\) |
N | \((1/4)^{N}\) | \((3/4)^{N}\) |
Methods for introgression of PGR
Several methods are available to introgress advantageous genetic variation into elite backgrounds. They include approaches based on classical plant breeding as well as genetic engineering. The most recent development is genome editing because it allows novel approaches of utilizing and characterizing plant genetic resources by way of neo-domestication, for example.
Backcrossing into elite germplasm
Although introgression based on phenotypic analysis has been used for a long time, it has been effective only with a simple genetic architecture such as dominant disease resistance genes. Steven Tanksley (Cornell University) promoted the use of a genotype-based rather than a phenotype-based approach using modern genomic techniques (S. Tanksley and Nelson, 1996). By using linkage maps, which are genetic maps where the location of markers are known, and a breeding technique called advanced backross QTL analysis, it is possible for the breeder to examine a subset of alleles in the genetic background of an elite cultivar (Figure 7). The main point of this method is to conduct a backcross, select against undesired properties as part of the prebreeding program and then to conduct a QTL analysis in the BC2 or BC3 generation to identify useful alleles for the breeding goals.
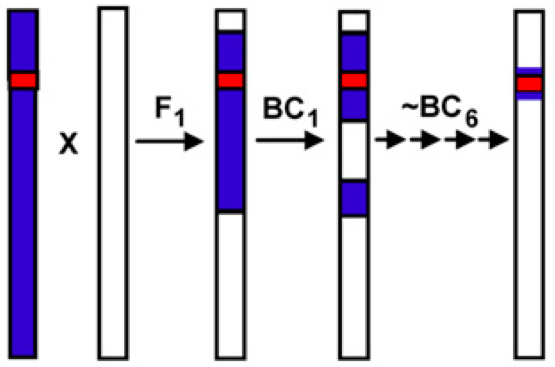
If a genetic marker is available that is linked to the allele (or gene) of interest, selection can be carried out based on the marker genotypes at an early stage of plant development, which makes it an efficient method for introgression. The use of genetic markers in the selection procedure is called marker-assisted selection (MAS). A marker that is linked with the desired phenotype is used for the selection rather than the phenotype itself.
Crossing a strategy for introgression
Although the general principle of backcrossing and introgression is simple, many crossing schemes are possible that may contribute to an optimization of the process. For example, instead of backcrossing with the same elite parent, the second cross may use the offspring of a cross of two different elite parents (Table 2). Since four parents are involved, such a cross is called four-way cross (4W).
Backcross | Four-way cross | |
---|---|---|
Season 1 | Resource x Elite A | Resource x Elite A |
Season 2 | (Resource x Elite A) x Elite A | (Resource x Elite A) x (Elite B x Elite C) |
After two generations of crossing, the progeny of a 4W cross contain 25% of exotic genetic variation as a regular backcross (Figure 8). Therefore, the exotic variation is contained three different elite backgrounds and for this reason is tested in a broader elite context, but can be realized in the same time span as a regular backcross.
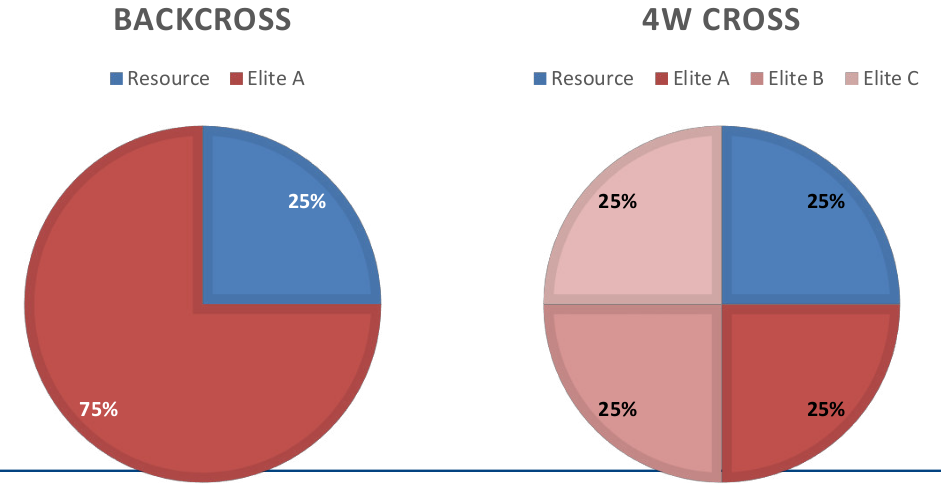
For this reason, a decision about the specific type of backcross needs to consider the objectives of the prebreeding program. In the project Genebank2.0, which aims to characterize the wheat genetic diversity of the IPK Genebank, multiple crossing strategies as shown in Figure 9 are currently being compared to answer the following questions:
- How many resources are required for each crossing strategy?
- How many elite parents (minimum and maximum numbers) should be used?
- Are there any cytoplasmatic effects of the different parents that may interfere with the nuclear genome of the resources?
These questions are addressed by computer simulations and experimental studies consisting of field trials.
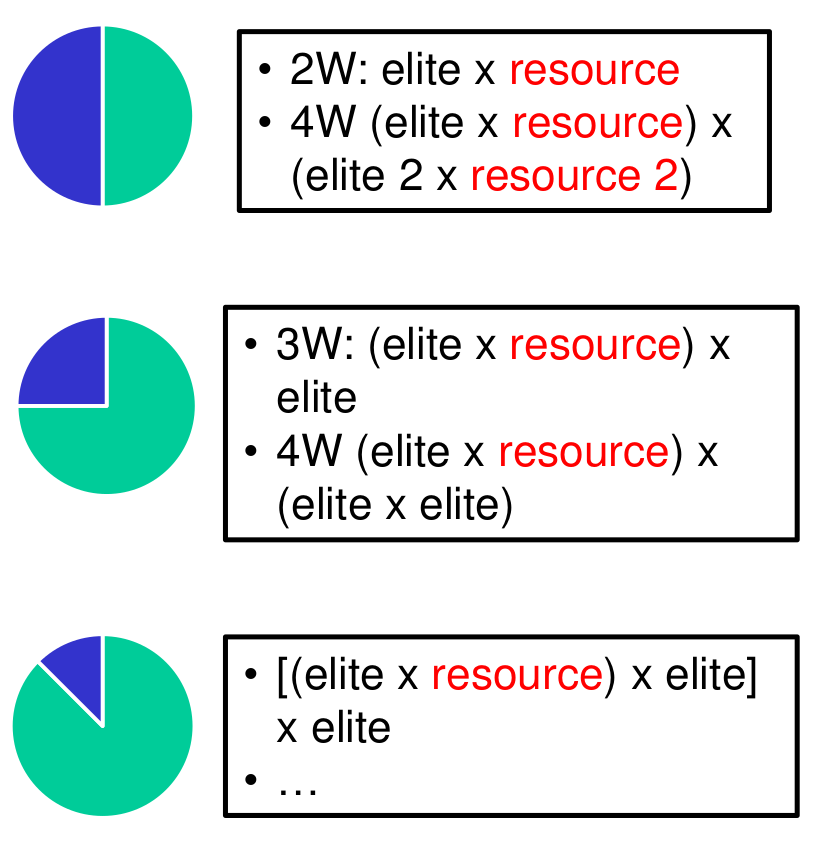
Pyramiding of multiple genes in a single genotype
Pyramiding is defined as the introgression of multiple genes with a strong positive effection on a phenotype into a population.
A variant of MAS is Marker-assisted recurrent selection (MARS). This method involves the crossing of selected individuals at each breeding cycle. By this approach, desirable alleles can be funneled into the breeding scheme from many different sources and combined into single elite lines.
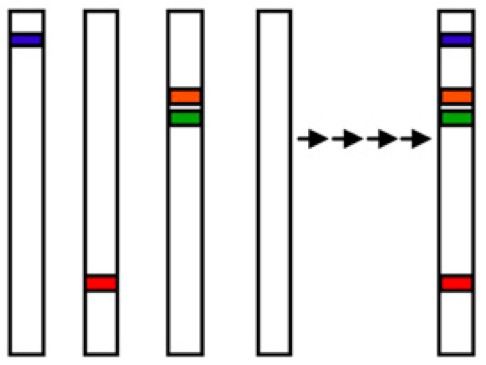
1 A more comprehensive overview of approaches to breed a wheat with multiple durable resistance and other (e.g., environmental) advantages is in (ayala_engineering_2024?)
The introgression of multiple QTLs into an elite variety is called pyramiding of QTLs (Figure 10). It can be used to create durable disease resistances by selecting two or more resistance genes against a pathogen. For example, a strong and durable resistance against rust in wheat can be achieved by combining partial but multiple resistance genes in elite varieties using MAS. Figure 11 shows an application of pyramiding in a current commercial wheat breading program. In this scheme, resistances agains four diseases (powdery mildew, leaf rust, eyespot disease and fusarium head blight) as well as a quality trait (grain protein content) are combined in four crosses. The goal is to combine the favorable (resistance-providing) alleles at as many resistance genes as possible and then backcross with the best advanced material to enrich the genes of interest (which are screened with markers) in the next generations.1
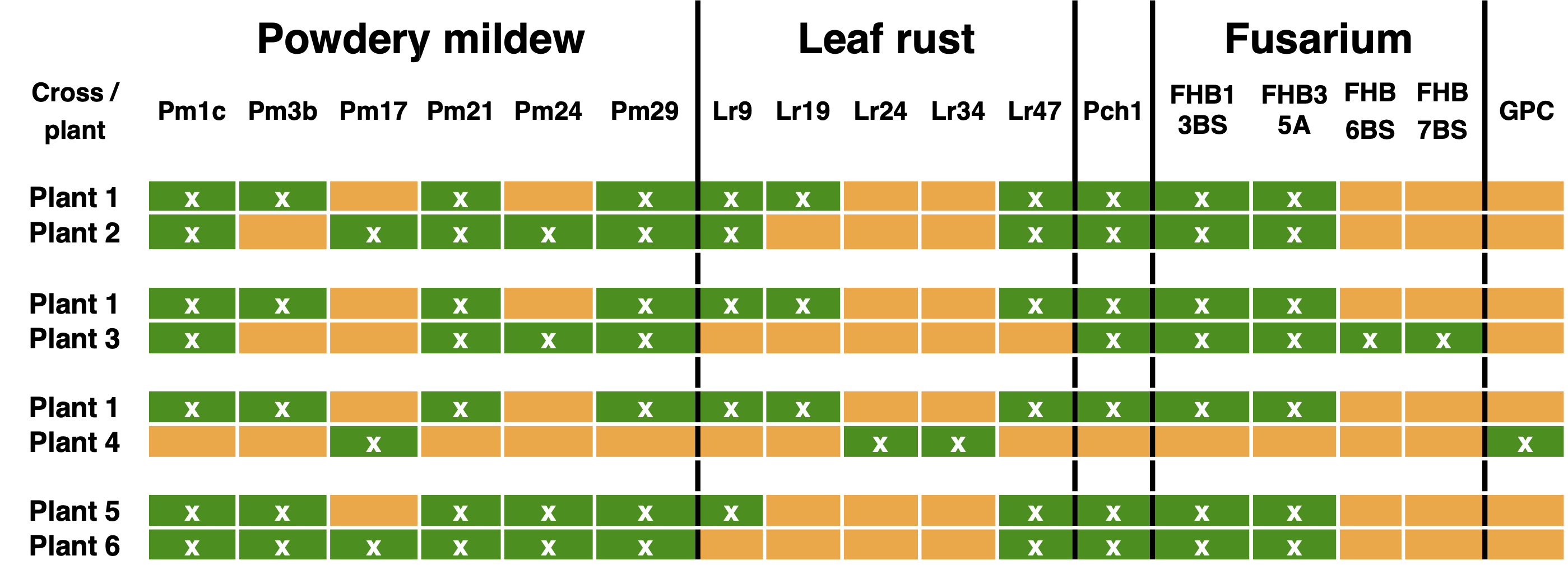
Many of the resistance alleles were initially derived from landraces or close relatives. For example the resistance allele against the eyespot disease, which is caused by the fungal pathogens Oculimanila acuformis and O. yallundae and may cause harvest losses of up to 40%, was isolated from Aegilops ventricosa (Figure 12).
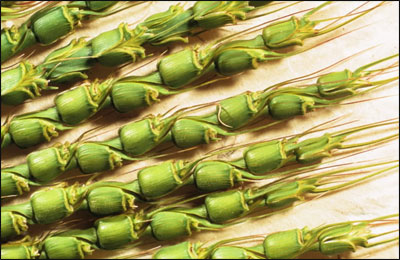
Genomic selection
Genome-wide or genomic selection is a form of marker-assisted selection and is under evaluation for the feasibility of incorporating desirable alleles at many loci that have small genetic effects when used individually. In this approach, breeding values can be predicted for individual lines in a test population based on phenotyping and whole-genome marker screens. The breeding value is the sum of the additive effects of alleles at multiple genes present in an individual genotype. Additive effects can be interpreted as regression coefficients of the genotypic value on the number of copies on a particular allele.2 These values can then be applied to progeny in a breeding population based on marker data only, without the need for phenotypic evaluation.
2 See any introductory book on quantitative genetics, e.g., Falconer and Mackay (1996).
The key principles of genomic prediction can be expressed with the following model. The basic genetic model considers the phenotypic value (\(P\)) of an indivudual as the sum of the additive (\(A\)), non-additive (i.e. dominance and epistatic) (\(NA\)) and the environmental values (\(E\)), \[\begin{equation} \label{eq:geneticmodel} P = A + NA + E \end{equation}\] The goal of genomic prediction is to predict the phenotypic vales from genome-wide genetic markers by constructing a model that accounts for all additive effects of genomic regions to which the genetic markers show a significant linkage. The prediction is achieved by a linear mixed model, which models the individual regression coefficients of each marker on the genotype using the following equation \[\begin{equation} \label{eq:blupmodel} \mathbf{y} = \mathbf{Z}\mu + \mathbf{X\beta} + \mathbf{e} \end{equation}\] where
- \(\textbf{y}=n\times 1\) is a vector of phenotypic values for a trait and individuals
- \(\textbf{Z}=\) the design matrix
- \(\mu=\) intercept
- \(\textbf{X}=n\times p\) matrix of marker genotypes
- \(n=\) Number of individuals phenotyped
- \(p=\) number of markers
- \(\mathbf{\beta}=\) vector of regression coefficients
- \(e=\) residual
The following numerical example shows an application of this equation.
\[\begin{equation} \label{eq:data} \begin{bmatrix} 100 \\ 107 \\ 102 \end{bmatrix} = \begin{bmatrix} 1 \\ 1 \\ 1 \end{bmatrix} \mathbf{\mu} + \begin{bmatrix} 2 & 0 & 2 \\ 2 & 0 & 0 \\ 0 & 2 & 2 \end{bmatrix} \mathbf{\beta} + \mathbf{\epsilon} \end{equation}\]The numbers in the \(\textbf{X}\) matrix are the allele dosages of allele \(A_1\), i.e. 2 indicates the genotype \(A_{1}A_{1}\), 1 the genotype \(A_1A_2\) and 0 the genotype \(A_2A_2\). In completely homozygous genotypes (i.e., doubled haploid lines), \(\textbf{X}\) contains only 0's and 2's.
To obtain the unknown parameters \(\mathbf{\mu}\), \(\mathbf{\beta}\) and \(\mathbf{\epsilon}\), an ordinary linear square (OLS) estimate of the regression coefficients have to be obtained using the following linear algebra theory3
3 A derivation of this equation can be found in any introductory textbook on linear algebra.
An application of this simple approach can be demonstrated in the following. Assume the following marker genotypes for the three individuals which have been phenotyped for a trait.
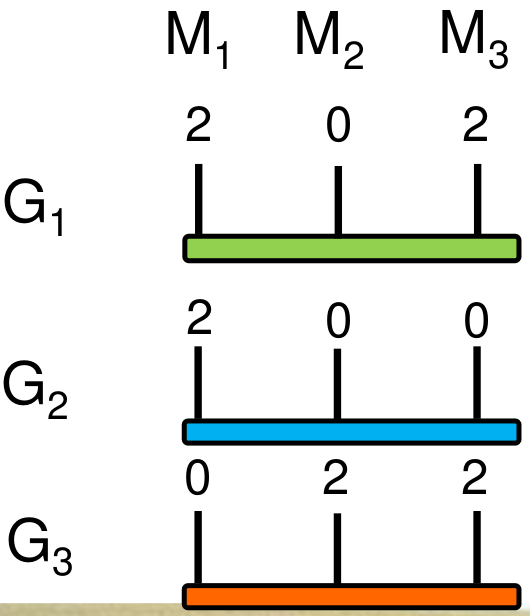
Using Equation\(\eqref{ols}\), the vector \(\mathbf{\beta}\) is estimated as
\[\begin{equation} \label{eq:gsexample} \mathbf{\beta} = \begin{bmatrix} -2 \\ 3 \\ 5 \end{bmatrix} \end{equation}\]The genomic estimated breeding values (GEBV), which is the predicted phenotype, is then calculated as
\[\begin{equation} \label{eq:gebv} GEBV = \sum_i^pX_i\beta_i \end{equation}\]For genotype \(G_{1}\) the GEBV is then the sum of \[2\times -2 + 0 \times 3 + 2 \times 5 = -4 + 0 + 10 = 6\] and estimated GEBVs of all three genotypes \(G_{1}, G_{2}, G_{3}\) in Equation (ref:eq:data) and Figure 13 are then
\[\begin{equation} \label{eq:gebvestimate} GEBV = \begin{bmatrix} G_{1} \\ G_{2} \\ G_{3} \end{bmatrix}= \begin{bmatrix} 6 \\ -4 \\ 16 \end{bmatrix} \end{equation}\]If vector \(\mathbf{\hat{\beta}}\) has been estimated for a training population, it can be used to predict the phenotype for any other population which only was genotyped, but not phenotyped. OLS estimates require \(n>p\) (i.e. a large training population), whereas in genomic prediction usually \(n \ll p\) because the number of markers is much larger than the number of phenotypic observations. In these cases, no OLS solution can be obtained, or estimates are unreliable.
To account for this problem, a variety of methods were developed that are classified into parametric, semi-parametric and non-parametric models. Parametric models assume normality, linearity and independent explanatory variables. However, these assumptions do not always hold and for this reason it is quite common that studies on the usefulness of genomic prediction in plant breeding compare prediction ability (and/or prediction accuraca) of different methods for genomic prediction using genome-wide marker sets (Figure 14).
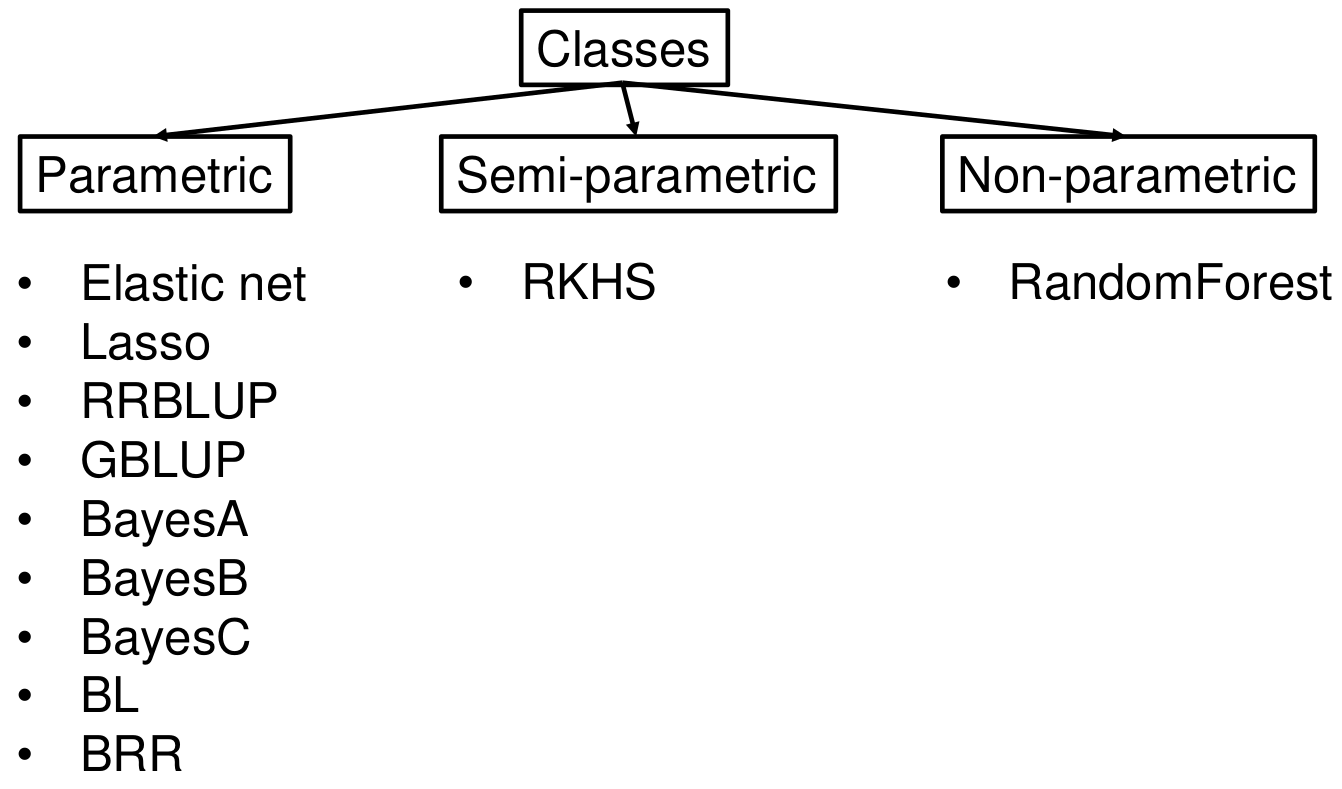
Nevertheless, modeling studies indicate that selection based on genomic prediction can lead to considerable increases in the rates of genetic gain by accelerating the breeding cycles (Heffner et al., 2009). In the oil palm, for example, this approach could lead to the release of improved germplasm after only 6 years as compared with the current time of 19 years.
Applications of genomic prediction in the context of plant genetic resources
Genomic prediction can be used to predict the phenotype of plant genetic resources that are genotyped but not phenotyped. In the context of plant genetic resources, genomic prediction has two main applications. First, the prediction of phenotypic variation in a large genebank collection using a smaller subset of accessions that have been both phenotyped and genotyped. The predicted phenotype can then be used to select genebank accessions that might be useful as parents in pre-breeding programmes or for other purposes. A second application is the use of genomic predictions to facilitate introgression of useful polygenic traits from exotic genetic resources into elite backgrounds through backcrossing or similar approaches. Two examples of the first application are presented in the following.
A set of 962 biomass sorghum accessions from a large genebank collection 34,844 accessions were selected by expert genebank curators to represent the phenotypic diversity of the material (Figure 15 A) (Yu et al., 2016). This reference set was then genotyped to obtain 340,496 SNPs and then the population structure was inferred (Figure 15 B).
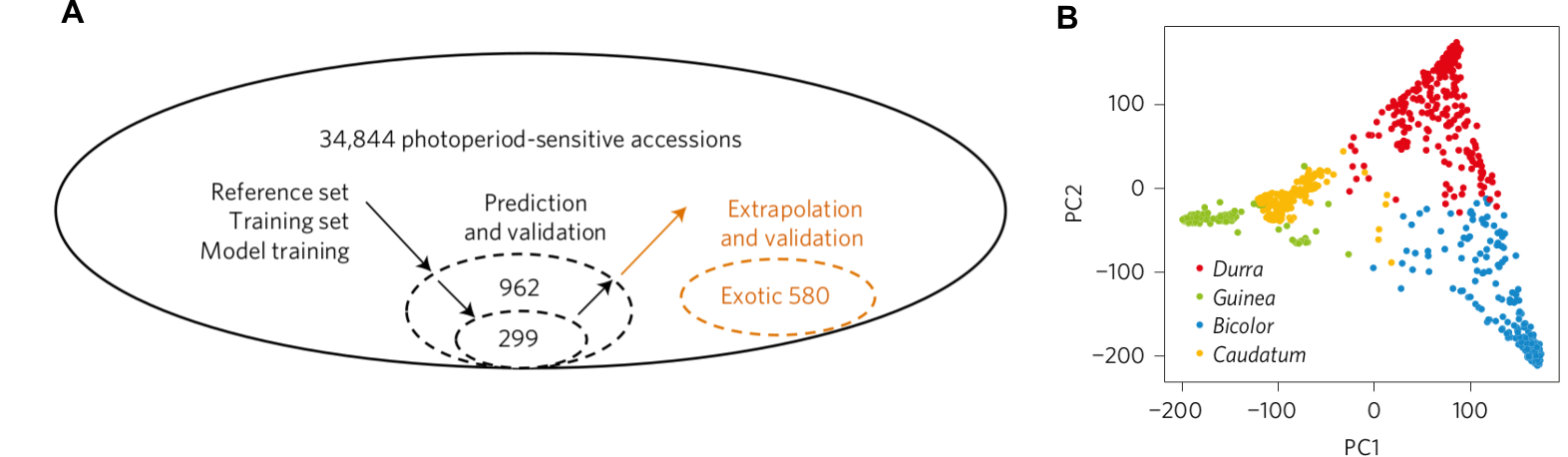
A subset of 299 accessions was selected as core collection to represent the diversity of the larger set and phenotypically characterized for several traits that also included biomass yield. Using the genotypic and phenotypic data of this material, genomic prediction models were trained. Their robustness was evaluated with a statistical method called cross-validation (Wikipedia), which consists of subsampling, model training using this subsample and prediction of the remaining sample. In addition, for independent confirmation of prediction quality, another set of 200 accessions selected and independently phenotyped. Using the genomic prediction model of the initial training set, trait values for this validation set were predicted and compared with the observed values to evaluate the robustness of the model (Figure 16). This comparison revealed a high prediction accuracy of genomic prediction, which indicates that genomic prediction is a useful approach for a cost-efficient characterization of plant genetic resources from genebanks.
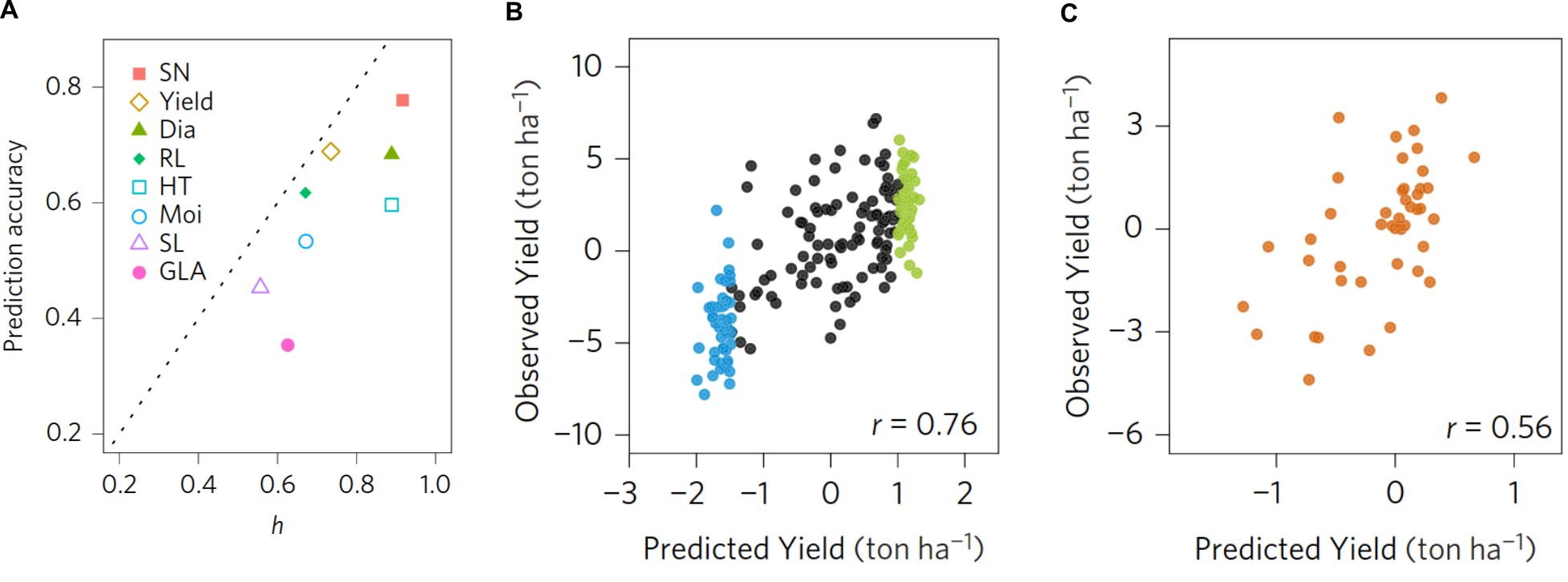
The second example is from the prediction of a of yield related traits in cauliflower Brassica oleracea var. botrytis (Thorwarth et al., 2018). The difference to the previous example is that this species is outcrossing and therefore shows a higher level of heterozygosity and phenotypic variation within genebank accessions. In addition, the phenotypic traits are more elaborate to characterize and usually smaller number of accessions can be phenotyped than with field crops. In the study a total of 174 randomly selected genebank accessions from two (USDA and IPK) genebanks were phenotyped for six curd-related traits at two locations (Heidfeldhof and Kleinhohenheim, both in Stuttgart, Germany) over three growing seasons. The accessions were genotyped resulting in 120,693 genotypes. Population structure analysis revealed five genetic groups in the material, which differed by their mean phenotypic traits such as flowering time (Figure 17).
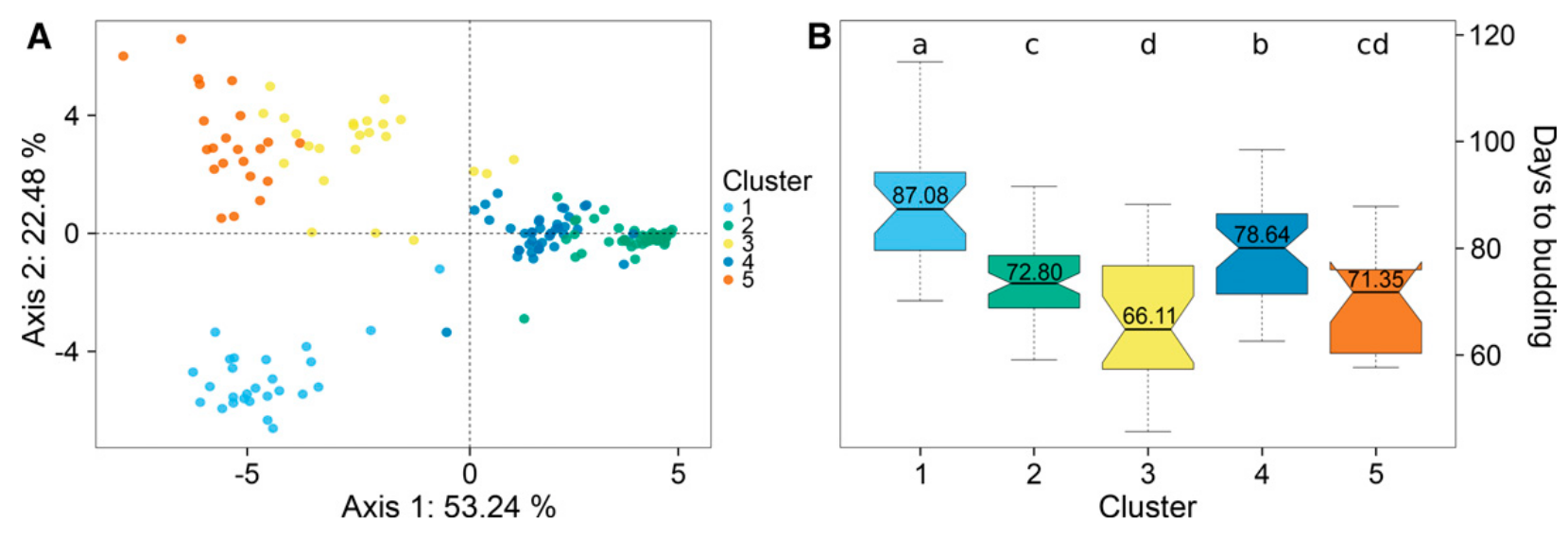
By combining genotypic and phenotypic data, various models of genomic prediction were trained and evaluated by cross-validation. Prediction abilities in the cross-validation ranged from 0.10 to 0.66 for the various traits (Table 3), and is little correlated to broad sense heritability. \(H^{2}\).
Trait | \(H^{2}\) | Prediction ability |
---|---|---|
Curd Width | 0.437 | 0.43 |
Cluster Width | 0.564 | 0.63 |
Number of Branches | 0.264 | 0.35 |
Apical Length | 0.111 | 0.12 |
Nearest Branch | 0.050 | 0.25 |
Number of Days | 0.943 | 0.57 |
A strategy for the use of genomic prediction for the introgression of exotic genetic variation into elite material was described by Gorjanc et al. (2016) for prebreeding programs of maize. In such a strategy, maize landraces are evaluated using genomic prediction approaches as described above to select a combination of landraces that enrich favorable alleles for polygenic traits to create a starting or pre-breeding germplasm (Figure 18). Such an optimized germplasm is then suifor crossing with elite lines of the same heterotic groups as the landraces, which then creates a bridging germplasm. The advanctage of such a bridging germplasm is that it contains a low proportion of exotic genomes thereby reducing deleterious genetic variation (genetic load) that may interfere with DH production and also linkage drag between favourable and undesired genetic variation. DH lines constructed from the bridging germplasm may then be used as donor lines for introgression into elite germplasm.
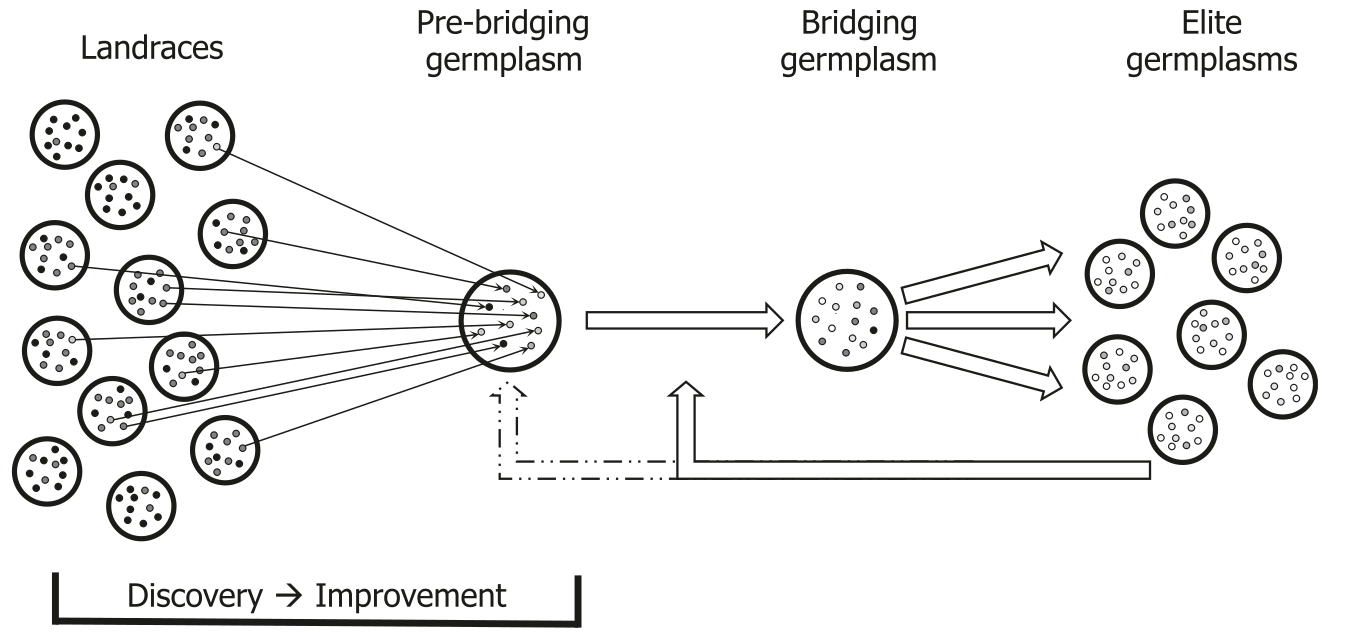
Exotic libraries as a source of genetic diversity
For traits with a simpler genetic architecture, so called libraries of lines with exotic introgressions are a useful alternative. Different types of populations such as F\(_2\) populations, backcross populations, recombinant inbred lines and advanced backcross lines between wild and cultivated species have been used to clone QTLs. However, such lines are frequently difficult to use for breeding because they contain large segments of wild material that may cause (partial) sterility. Zamir suggested to use exotic libraries or introgression libraries, which are essentially introgression lines that are produced by backcrossing and self-fertilization for up to 10 generations (Zamir, 2001).
The principle of creating an exotic library is shown in Figure 19. Different lines of an exotic library carry a single, defined (by marker analysis) segment from a wild variety in an otherwise elite background.
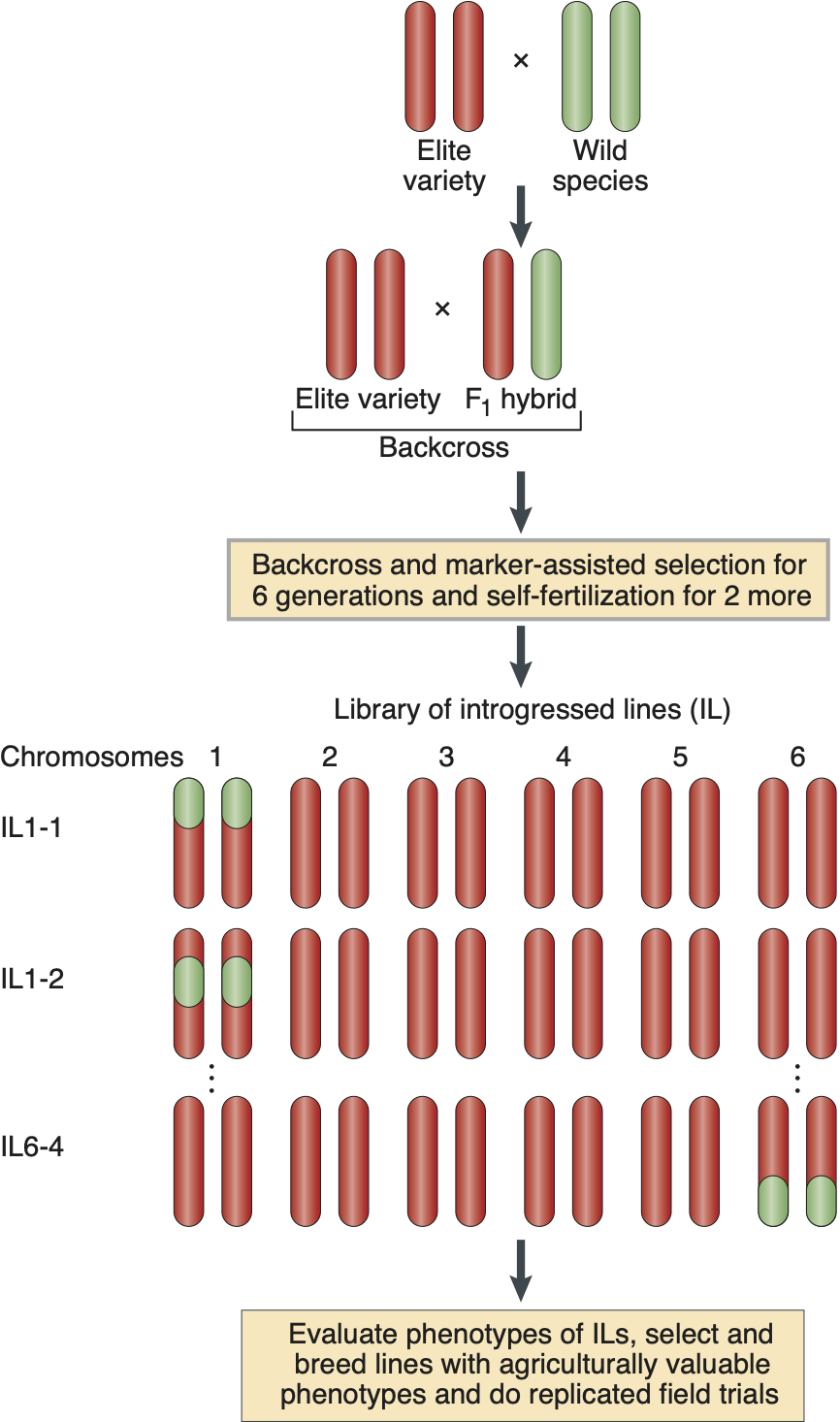
Creating the lines consists of the following steps:
- Cross a wild species with an elite variety
- Backcross the F\(_1\) hybrid with the elite variety
- Repeat this step for 6 generations. In each generation, the proportion of the wild species is reduced by 50% on average in each line.
- Trace chromosome segments by genotyping with markers the differentiate wild and elite chromosomes.
- After six generations, isolate independent plants which are heterozygous for different segments of the wild genome.
- Self-fertilize the selected lines for one to several generations to make the wild chromosome segments homozygous.
- Screen the library for traits of agricultural importance.
An actual example of the changes in the genome of a IL library in tomato is shown in Figure 20.
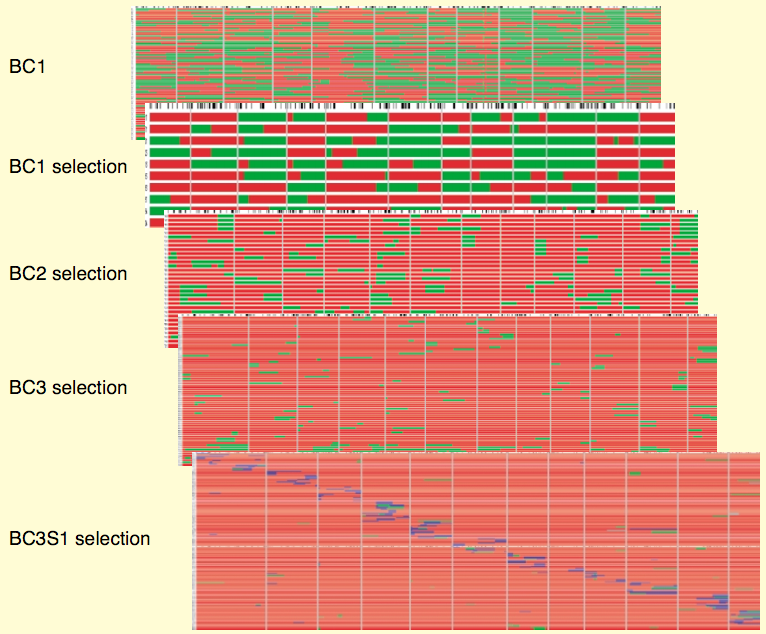
Statistical power of backcrosses
The statistical power of advanced backcrosses to detect QTLs was investigated by Tanksley and Nelson in computer simulations S. Tanksley and Nelson (1996). The expected decay of the average length of introgressed fragments is shown in Figure 21 in comparison to self fertilization over several generations. In both cases, an F\(_1\) population is considered, which was either backcrossed with one of the parents or self-fertilized.
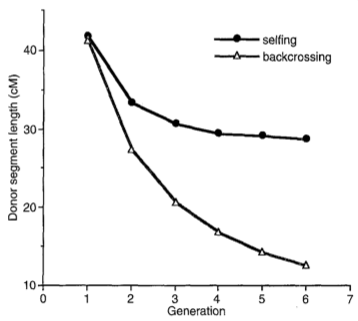
They also compared the power to detect QTLs in comparison to recombinant inbred lines obtained through repeated self-fertilization of an F\(_1\) population and found that it depends on the genetic architecture of the QTLs. If QTLs are purely additive, recombinant inbred lines (RILs) are more powerful. However, if donor QTLs are additive in the presence of another, unlinked QTL with a recessive donor allele, advanced backcrossing is more powerful (Figure 22).
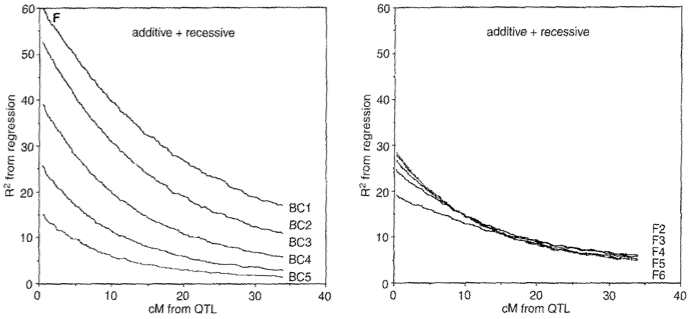
Advantages of backcrossed lines
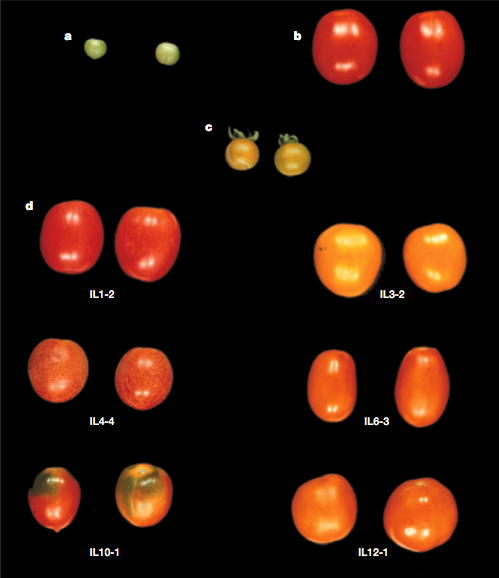
The total time of creating an exotic library takes about ten generations. Once the libraries have been generated, they have the following characteristics and uses:
- Reduced sterility problems, because the ILs resemble the cultivated variety.
- Epistatic effects due to other interactions within the wild species genome are reduced.
- All phenotypic variation is caused by short introgressed segments; hence the statistical power to detect small effects is increased.
- Exotic libraries are sand can be used by many research groups.
- Heterotic effects can be tested by crosses to different tester lines.
- QTLs can be mapped to small intervals by further backcrossing of selected ILs.
Tomato ILs that increase yield
Zamir and colleagues produced an introgression library with the close relatives Lycopersicon pennellii identified three ILs, that increased yield by up to 50% under both irrigated and drought conditions Gur and Zamir (2004). They compared yield increase in three ILs and in an IL where all three segments were combined into a single line (IL789) in homozygous and heterozygous condition with the the parental elite variety M82 (Figure 24).
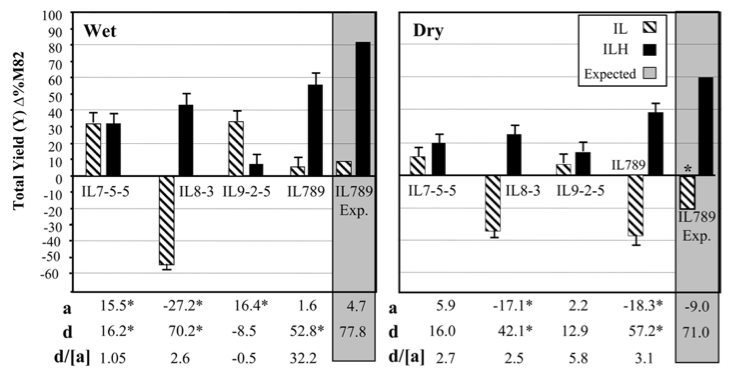
Examples of introgressions into modern varieties
There are several examples of the introgression of exotic genes into modern material by classical breeding methods Zamir (2001). The list of examples for the targeted introgression of exotic genetic diversity is growing very rapidly.
Wheat
- About 30 disease resistance genes were introgressed in wheat from wild relatives.
- Introgression of a region of chromosome 1B with the homologous region from rye, Secale cereale, led to higher hields in optimal and stress environments.
- Introgression of a rust resistance gene (Lr19) from the tall wheat grass Agropyron elongatum increased yield.
- Introgression of a high-grain protein QTL from Triticum dicoccoides (wild emmer wheat) improves the quality of pasta made from wheats with the QTL.
Tomato
- Modern commercial hybrids contain different combinations of 15 independently introgressed disease resistance genes that originated from different wild resources.
- Various QTL that improve fruit quality were introgressed.
- The gene \(B\) increases the level of provitamin A (\(\beta\) carotene) in the fruit by more than 15-fold. It was introgressed from the wild species Lycopersicon pennellii.
Rice
- Resistance genes for more than seven pathogen diseases were introgressed into tomato.
- Many yield QTLs for different environments were identified and will soon be introgressed into modern varieties.
Maize
During the domestication and breeding of maize, many important traits seem to have been lost. After identification of the causative mutations, markers are available that may be used to introgress the phenotype into modern varieties by marker assisted selection or genetic engineering.
A mutation in Lycopene \(\epsilon\)-cyclase leads to a reduced carotenoid content Harjes et al. (2008). The causative mutation was discovered and shown to result from a transposon insertion into the gene. Therefore, by using marker-assisted selection, MAS, modern varieties can be biofortified.
Another example concerns variation in seed oil content in maize kernels, which is due to a single amino acid mutation in the diacylglycerol transferase (DGAT) gene Zheng et al. (2008). The QTL was mapped and cloned using introgression libraries and the phenotypic effect on various seed traits was shown (Table 4).
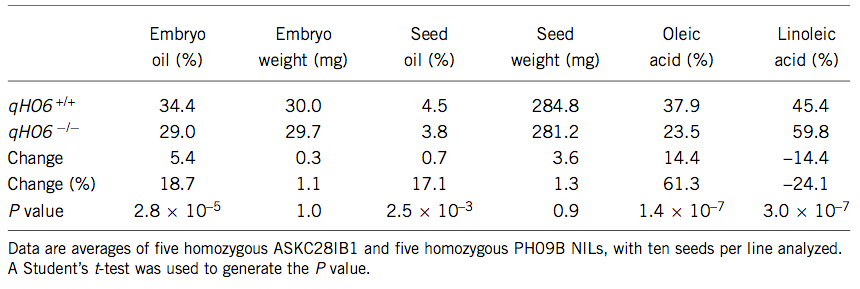
An evolutionary analysis indicated that a single amino acid mutation is sufficient to create the observed phenotype and it originated sometimes during maize domestication or breeding because the high-oil phenotype is ancestral (Figure 25)..
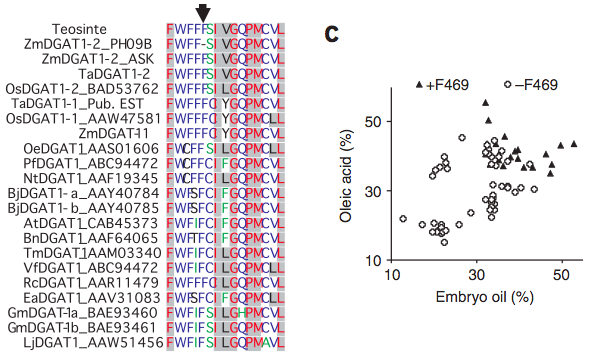
Introgression by genetic engineering
Another approach is the introduction of exotic genes and new genetic variation by genetic engineering (Figure 26). This approach has the advantages that it is faster than backcrossing and that only the desired gene is introgressed. However, it should also be noted that a certain degree of backcrossing is still necessary to produce commercial varieties.
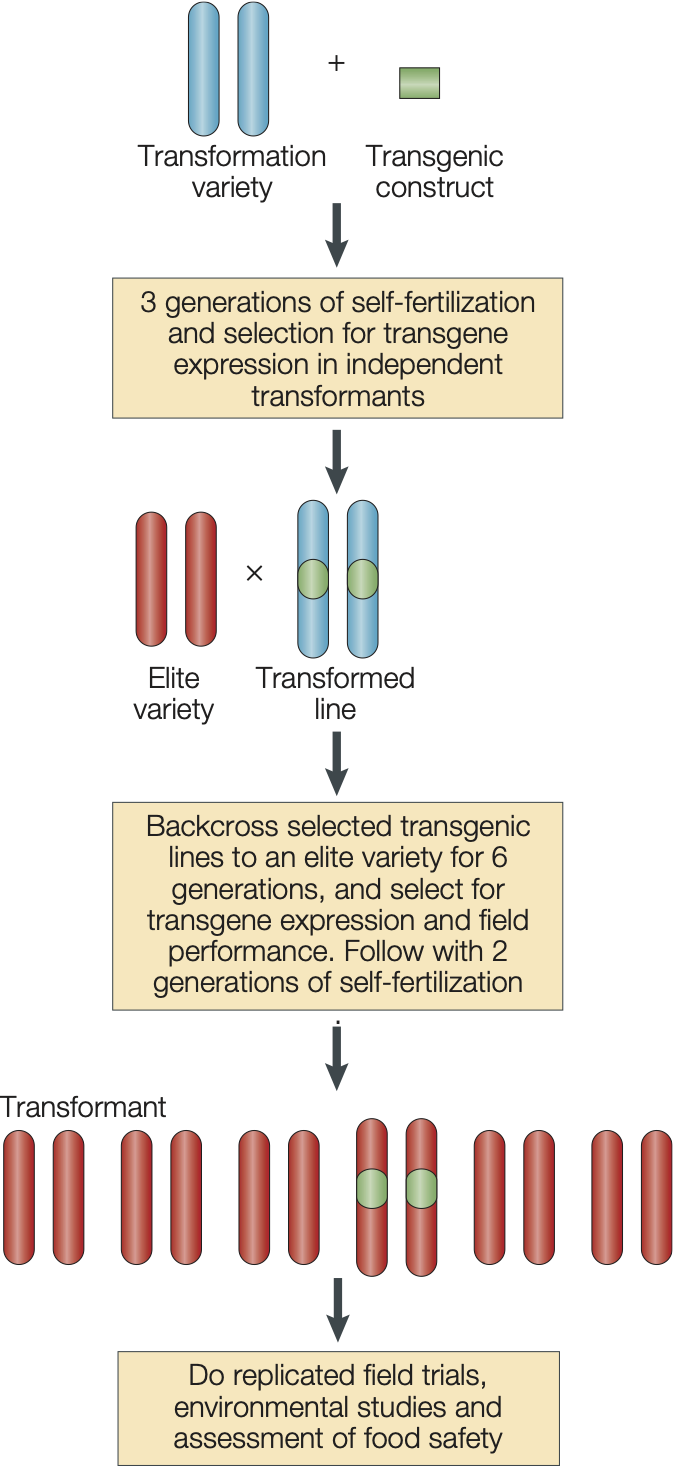
Restoring a lost trait: Exudates in maize
Plants often emit volatile compounds when they are attacked by herbivorous insects. The volatiles attract natural enemies of insects. Maize emits (E)-\(\beta\)-caryophyllene, which attracts entomopathogenic nematodes that infect and kill, for example, the western corn rootworm, which is an important pest of maize. Most North American maize varieties have lost this ability.
Volatile emission was restored by introducing a (E)-\(\beta\)-caryophyllene synthase gene from oregano (Degenhardt et al., 2009). In rootworm-infected plots, the transformed varieties had significantly less damage than the untransformed, non-emitting lines, because significantly fewer nematodes were attracted to the plants (Figure 27).
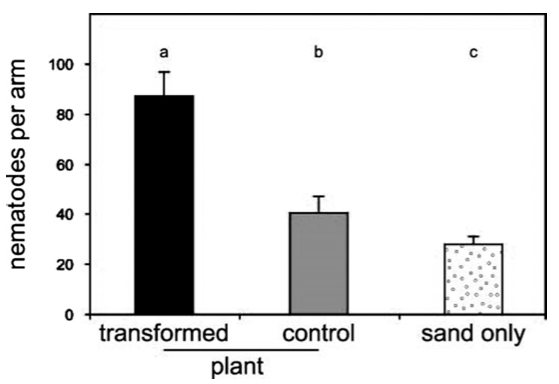
It is to be expected that many more of such examples will be discovered in the future because such genes are not usually selected during the plant breeding process and may therefore have been lost during the breeding history.
Resistance genes in potato
One recent example of a GMO introduction is the creation of a potato variety (Fortuna) by BASF Plant Science that is resistant against Phytophtora infestans. It was created by introducing two disease resistance genes from the Mexican wild potato Solanum bulbocastanum. This variety was brought into the deregulation process, which was stopped in 2013 due to the ongoing criticism of genetic engineering in plants. The experiment was repeated in a public research institution and demonstrates the power of such a transgenic approach to create pathogen-resistant plants (Figure 28).
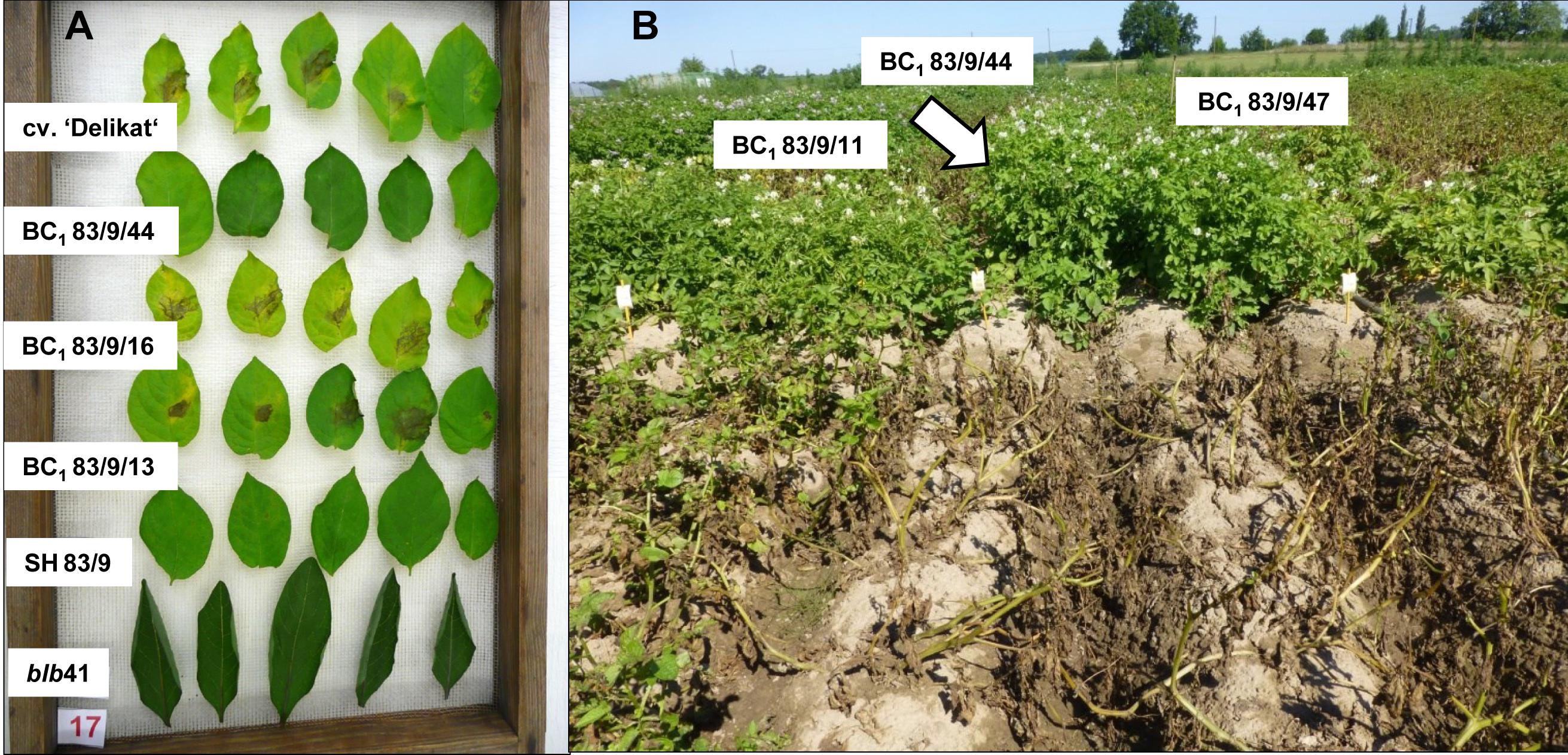
Cisgenic versus transgenic approaches
In response to the strong criticism of transgenic genetic modification, the concept of cisgenesis (Jacobsen and Schouten, 2009) was developed. It is the introduction of genetic variation using genetic engineering, but where the source is a close relative of gene pool 1 or gene pool 2 in the sense of Harlan and Wet (1971), i.e., from species that can be crossed with the target species. Differences between classical breeding, cisgenic genetic modification and transgenic transformation is shown in Table 5.
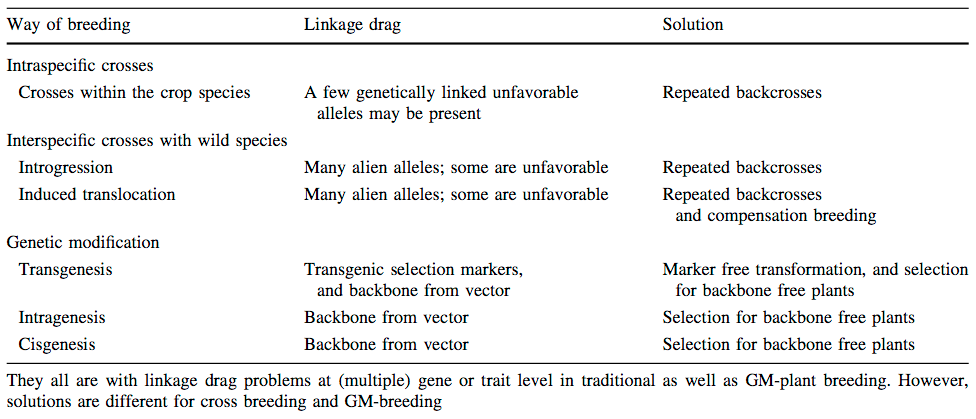
Cisgenesis is driven by two important technical developments:
- Marker free transformation without linkage drag of antibiotic resistance is routine for many generatively propagated crops but also for vegetatively propagated crops.
- Modern genomics via advanced gene cloning techniques, whole genome sequencing and bioinformatics.
Both are stimulating molecular isolation and insertion of many plant genes, called cisgenes, allowing variety improvement with only natural alleles from the breeders gene pool.
The key aspect is what concerns a transgene and what a cisgene as shown in Table 6. The important aspect of this classification is that they are proposed to be differentially regulated. However, current regulation differentiates cis- and transgenesis only with respect to biological safety (Cartagena protocol), but not with respect to regulation for release into the environment (Directive 2001/18/EC), which is relevant for conducting field trials or use in agriculture.
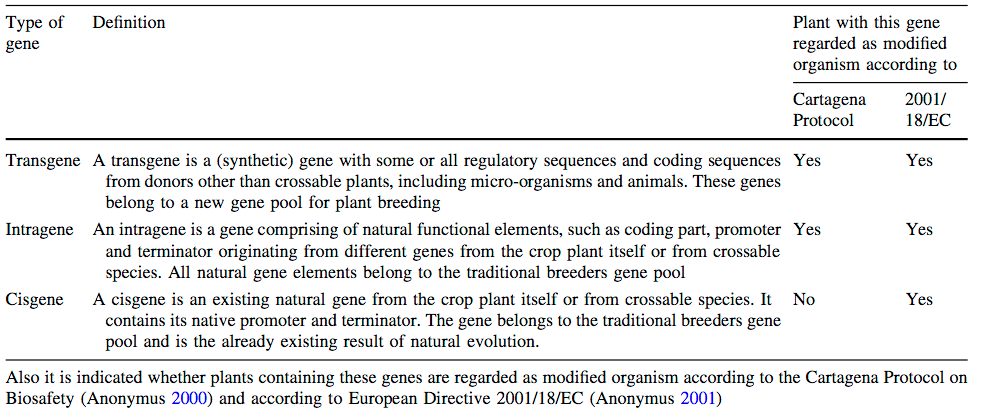
Genome Editing
The possibility of specifically modifying genes in plants is a new development in plant biotechnology. This technique is called genome editing or new breeding technologies and has become known as CRISPR/Cas9 in recent years, although this possibility has been possible in principle for about 20 years. However, because genome editing with CRISPR/Cas9 has moved strongly into the public focus and this method offers many advantages compared to previous methods, it will be presented here.
Essentially, genome editing with CRISPR/Cas9 is based on a series of discoveries about the immune system of bacteria to defend themselves against viruses. The key invention of Emmanuelle Charpentier and Jennifer Doudna is based on the production of a so-called guide RNA, which binds to a defined site in the genome and to which the Cas9 enzyme, an endonuclease, binds at the same time. This enzyme then generates a cut at this site, i.e. a double-strand break. Such double-strand breaks in DNA occur naturally with a certain frequency or are induced, e.g. by solar irradiation and the UV component contained therein. Subsequently, the repair systems of the cell recognize these breaks and repair them. Because these repair mechanisms do not work perfectly, new mutations (point mutations or small insertions/deletions) are generated with a certain frequency during repair. For the repair system of the cell, it makes no difference whether this double-strand break occurred naturally or was artificially generated. The newly generated mutations can subsequently be tested to determine whether they affect the function of the regulatory sequence (e.g. enhancer or promoter) or encoded gene contained in the edited one and thus generate a new phenotype. Shortly after the evolution of the CRISPR/Cas9 system, the potential of this method was recognized and many new variants were invented.
One important innovation is prime editing. With this method it is no longer necessary to create a double strand break, and instead bases can be directly exchanged by an appropriate template. The advantage of such an approach is that the resulting mutations no longer depend on chance, as in the natural repair system, but can be specifically designed.
Although genome editing has now been carried out in more than 40 plant species as a proof of concept, it is still far from being used as a standard method in breeding because the efficiencies in transformation, regeneration and mutagenesis are usually quite low. Exceptions are model organisms like Arabidopsis thaliana, in which genome editing can be applied fairly easily. However, due to the great importance of this method, it can be assumed that this will change quickly, which is why very high hopes are placed in genome editing as an important building block for the adaptation of agriculture to climate change and in food security.
Genome editing and plant genetic resources
In the context of plant genetic resources, genome editing is of interest in several respects. On the one hand, genome editing allows the reconstruction of beneficial or useful mutations discovered in plant genetic resources so that backcrossing is not necessary and linkage drag is significantly reduced. Complementarily, alleles present in cultivated plants (e.g. in domestication or improvement genes) can be generated in genetic resources such as wild relatives or landraces, so that re- or de novo domestication is possible.
Examples of these applications will be shown below.
Re-domestiation of landraces
As an example of the application of genome editing shall be the editing of a landrace variety of rice, where several genes were knocked out with the help of . The gene HTD1 controls plant height. In an African rice landrace, HTD1 was knocked out by genome editing to produce shorter plants to reduce lodging. In addition, three evolutionarily related and functionally similar genes (GS3, GW2 and GN1A) were edited with a multiplex CRISPR/Cas9 construct to affect and increase seed size.
In both cases, genome editing was very successful, and both plant height and seed size were successfully increased (Figure 29). As a result the overall yield of the landraces can be easily adjusted and brought to a level that may allow them to compete with modern, improved varieties, while they maintain many advantages of landrace varieties.
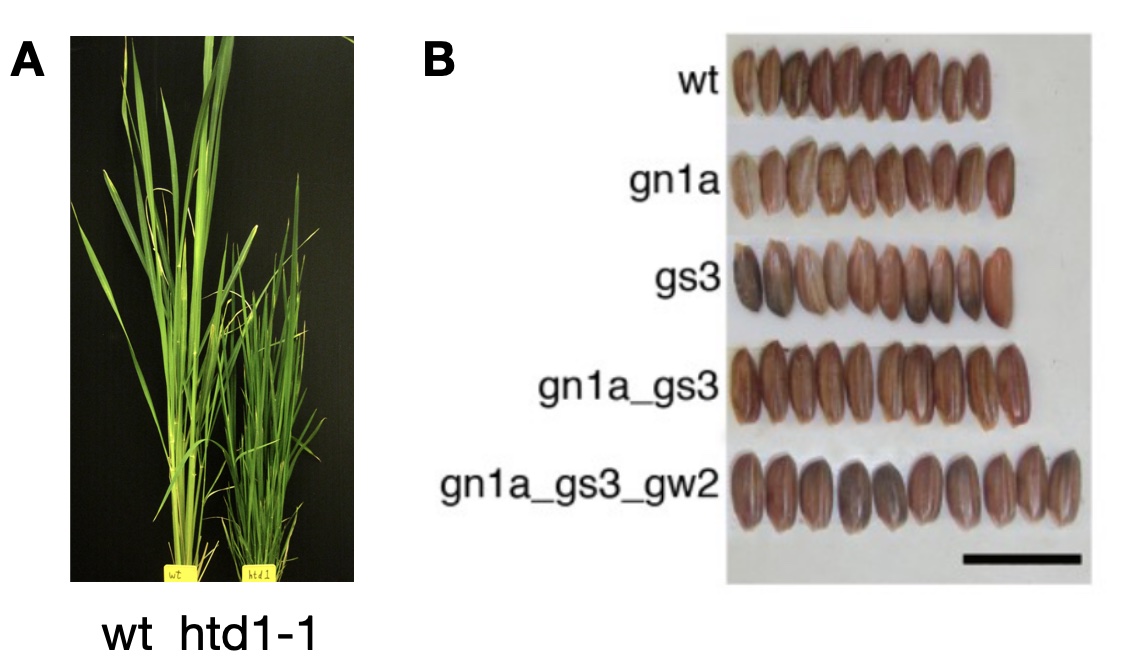
Neodomestication of wild crop relatives
In addition to the improvement of landraces, genome editing also has shown its potential in the rapid domestication of wild crop relatives, which is also called neo-domestication.
One example is the domestication of wild tomato Solanum pimpinellifolium by editing multiple well-known domestication genes Li et al. (2018). For the neodomestication of wild tomato, five genes were domesticated. They include genes that regulate the plant architecture and the synchronicity of fruit ripenin (SP), sensitivity to day length (SP5G), fruit size (SICLV3 and SIWUS), and vitamin C level (SIGGP1). The domesticated alleles of all of these genes are knock outs of gene function, and for this reason, they were easy to alter by genome editing.
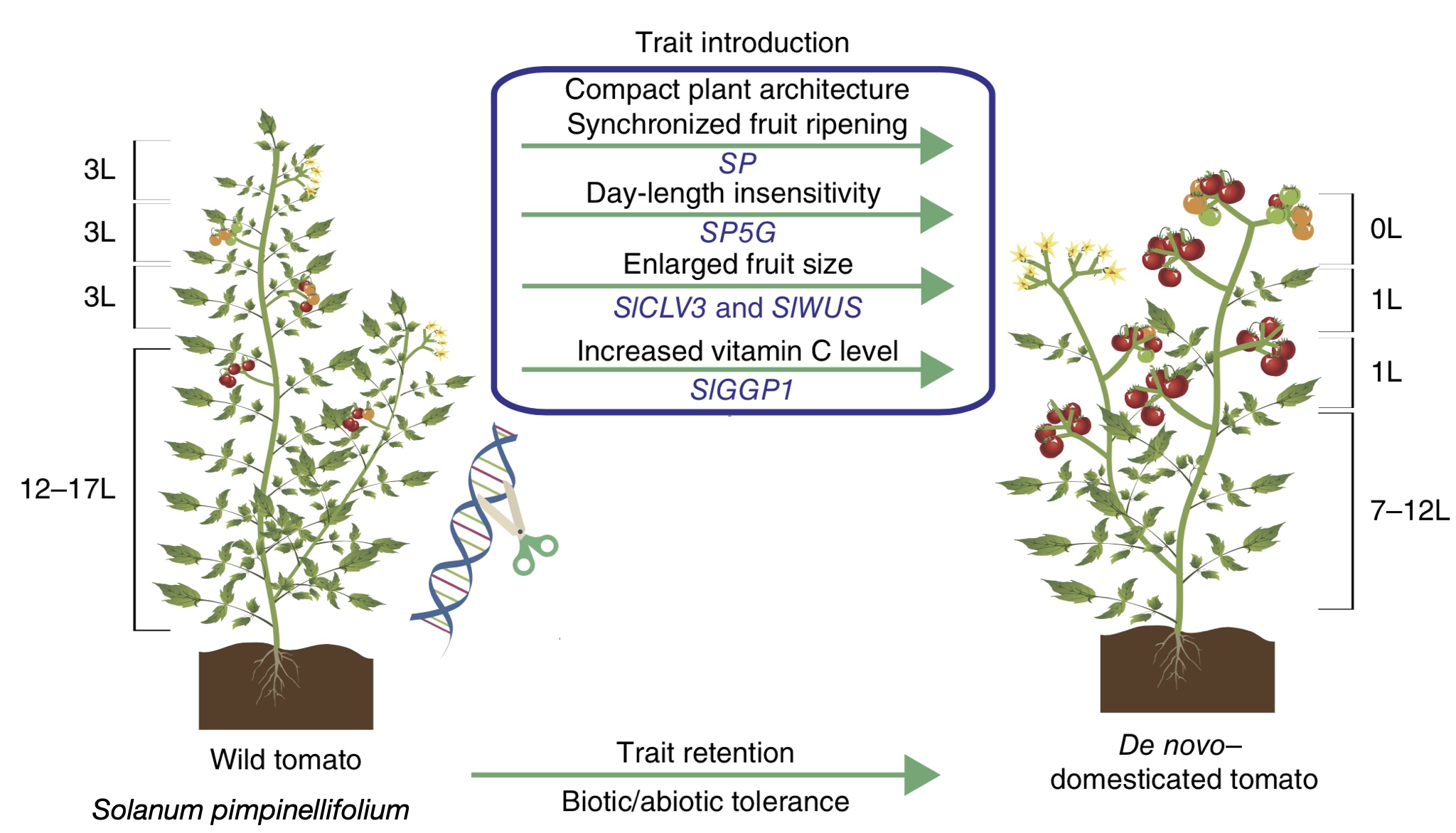
A key advantage of neo-domestication is that advantageous traits of wild relatives are preserved. For example, in the example of the wild tomato, the hypersensitive response to inoculation with pathogenic bacteria is preserved in gene edited plants, whereas the reaction missing in modern cultivars that leads to increased susceptibility to disease (Figure 31).
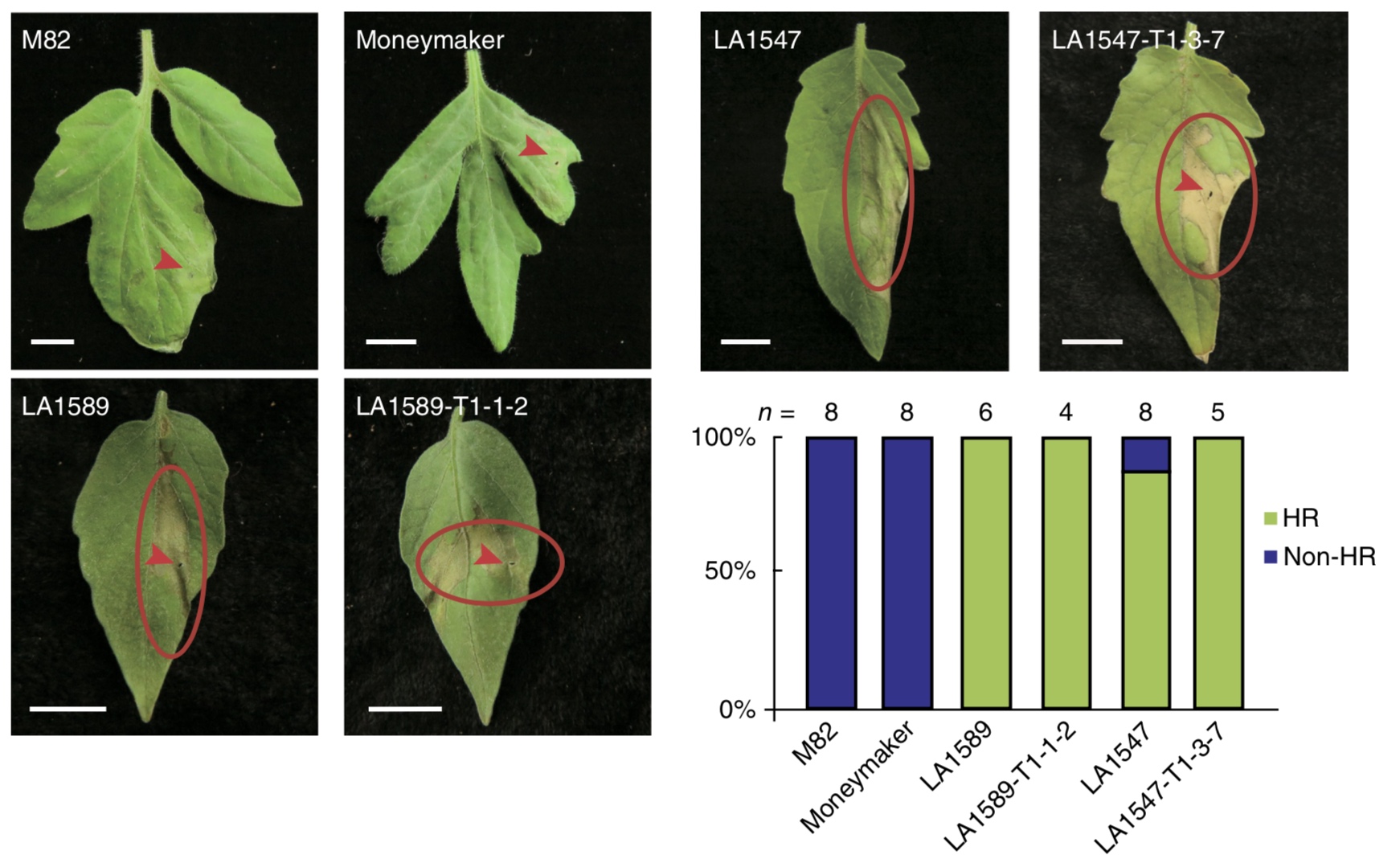
Another advantage of neo-domestication is that plants that have been commercially used for fruit production can be improved and optimized for commercial production by the targeted introduction of new mutations Lemmon et al. (2018). By genome editing the same domestication gene SELF-PRUNING 5G (SP5G) as in S. pimpinellifolium, the function of the gene was altered in a way that the genome edited lines had 50% more fruits, which makes them more interesting and relevant for commercial production (Figure 32).
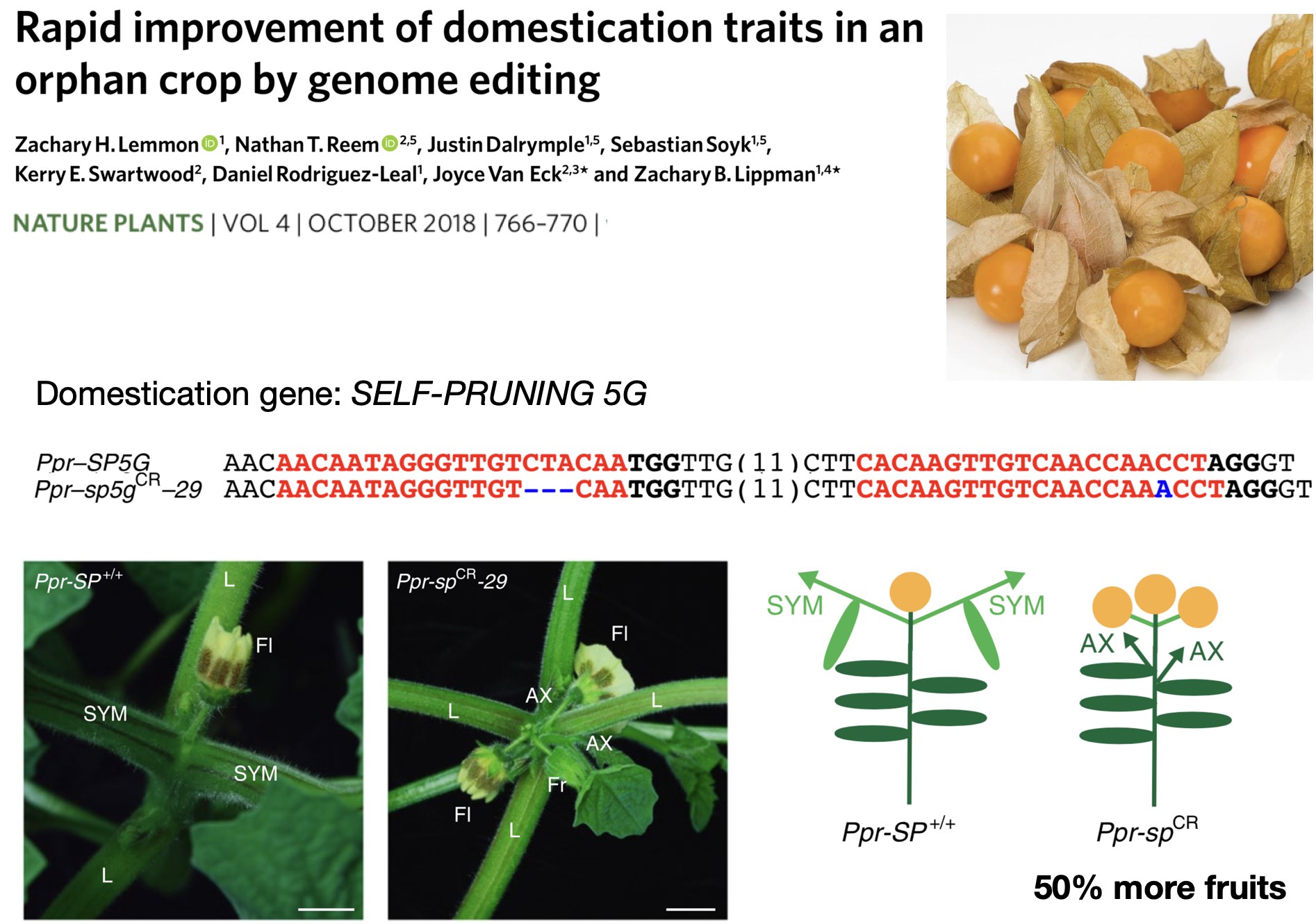
The ease by which plants can be genome edited has raised great expectations into this technology. The number of examples of genome edited plants with altered phenotypes is increasing rapidly and will lead to a new consideration of the value of plant genetic resources. For example, PGR will may achieve an additional role as donors of information about useful genetic variation using GWAS. It may suffice to use the information from PGR and genome edit candidate genes in elite varieties directly without the need of introgression.
Key concepts
\(\square\) Prebreeding | \(\square\) Transgressive segregation | \(\square\) Introgression breeding |
\(\square\) Backcrossing breeding | \(\square\) Genomic prediction and genomic selection | \(\square\) Pyramiding |
\(\square\) Marker-assisted selection (MAS) | \(\square\) Four-way cross | \(\square\) Genomic estimated breeding value |
\(\square\) Introgression libraries | \(\square\) Genome editing | \(\square\) Neodomestication |
Summary
- Various breeding approaches are available to facilitate the utilization of plant genetic resources.
- The plant breeding innovation cycle requires the constant introgression of new genetic diversity.
- The introgression of exotic genetic diversity aims to improve traits in elite material, but also is accompanied by some negative effects such as hybrid incompatibility, sterility or linkage drag.
- The most important methods are backdrossing, pyramiding, and genetid engineering.
- For prebreeding purposes, it appears to be more efficient to evaluate exotic sources for their suitability as crossing parents than to screen large segregating crosses with elite parents.
- Backcrossing is a suimethod for introgression of exotic diversity into one or multiple elite backgrounds.
- Pyramiding is the recombination of ^multiple alleles into a single elite genotype.
- Genomic selection is a suiselection method for complex traits and allows to predict the phenotype of genetic resources and is a breeding method for the introgression of such traits.
- Introgression libraries separate QTLs from their genetic background and allows to analyse their additive effects in different (elite) backgrounds with high statistical power and also allow their use in the breeding.
- Genetic engineering is a method to introgress genetic diversity from very diverse genetic sources and avoids some problems such as linkage drag.
- Genome editing is a new and revolutionary approach to utilize plant genetic resources for plant breeding. In particular, the potential arises from the mutation of individual genes to either neo-domesticate crop wild relatives or to edit a few genes for introducing derived propertis such as high yield or disease resistance while maintaining favorable characteristics of the exotic germplasm (i.e., certain adaptations or a high genetic diversity).
Further reading
- Feuillet et al. (2008) - A good overview over using exotic genetic resources in wheat
- Tester and Langridge (2010) - A review of the implications of plant breeding on food security.
- Longin and Reif (2014) - A suggestion on how to use genetic resources in wheat breeding
- Moose and Mumm (2008) - A nontechnical introduction into plant breeding and methods for introgression of exotic diversity
- Gao (2021) - A comprehensive vision of using genome editing in plant improvement including neo-domestication
Review questions
- What is a plausible genetic explanation for transgressive segregation, which may justify the introgression of exotic genetic diversity in elite breeding material?
- What are the relative advantages and disadvantages of backcrossing versus pyramiding for the introgression of exotic genetic diversity into elite breeding material?
- Can you think of a strategy to reduce linkage drag in classical plant breeding approaches such as backcrossing?
- Why is it advisable to develop bridging germplasm in prebreeding instead of directly funnel exotic genetic diversity into elite breeding programs?
- Do you think that scientific reasons or the current regulatory landscape for genetic engineering of plants limits the application of genetic engineering in the utilization of plant genetic resources?
- Explain the key principle of genomic selection and why it is a valuable approach in the utilization of plant genetic resources.
- All breeding strategies presented assume that a small proportion of exotic germplasm is introgressed into elite germplasm. Can you also think of scenarios, in which the opposite makes sense?
- One criticism of the cisgenic approach as opposed to a transgenic approach was that it is not a support for a transgenic approach in plant breeding and PGR utilization, but in fact may weaken the broad acceptance of genetically engineered plants. Can you find some arguments that would support this criticism?
- Can you think of a scenario in which genome editing may make the utilization of plant genetic resources in plant breeding obsolete, or at least opens avenues for different uses than how they are used now?
In-class exercises
To be added
Problems
To be added