Allele mining
Motivation
The identification of new and useful genetic variation is one of the primary goals and functions of both ex situ and in situ gene banks, as well as the diversity that is present in world wide breeding programs. The identification and subsequent utilitation of genetic variation is frequently called allele mining, which is defined as the identification of useful genetic variation ind exotic germplasm (wild relatives and landraces) as resource for improving modern elite varieties.
The purpose of this lesson is to demonstrate the application of allele mining. A key goal is to raise the awareness that knowledge of the population genetic history of a crop and of its geographic distribution is highly useful for allele mining because in combination with environmental parameters it allows to identify adaptive variation in known genes or novel genes of interest.
Learning goals
- Understand how allele mining is used to identify and utilize useful genetic variation from exotic germplasm (wild relatives and landraces) for improving modern elite varieties .
- Describe and contrast core-collection sampling, environmental association mapping, targeted allele mining of known genes, tests of selection, and population-genetic modelling as strategies for uncovering adaptive variation .
- Outline the Focused Identification of Germplasm Strategy (FIGS), including the integration of phenotypic, passport (geographic/ecological), and climatic data, and the use of multivariate analyses to predict adaptive traits .
- Describe how sequence-based diversity analyses can reveal signatures of positive selection and prioritize candidate resistance alleles in plant genetic resources.
The use of PGR for breeding of improved varieties
We define genetic resources as exotic (non-native) or old landraces and the crop wild relatives of modern crops that evolved in and adapted to specific environments and agricultural systems. The resources can be crossed into current breeding populations for the continuous improvement of elite material. Genetic resources can may also be useful as sources for new traits to meet new challenges and requirements in crop cultivation:
- Adaptation to global change
- Create varieties that tolerate higher temperatures and longer periods of low precipitation or periods of flooding, for example
- Opening of new regions for cultivation
- Expand the cultivation range, for example in cooler regions such as Northern Europe by improving cold tolerance (of maize, for example)
- New uses
- Develop new uses such as bioenergy crops for biogas production by selecting varieties with a later flowering time or a larger growth potential for higher biomass.
The main challenge is to identify useful genetic variation that allows to achieve these goals and to separate it from disadvantageous variation during the introgression into elite material by classical breeding or genetic engineering.
Diversity in countries of origin
In many cases the major crop plants originated in different countries than those in which they are mainly cultivated (Figure 1). Since crop genetic diversity in the areas of domestication tends to be high than in other regions, chances that useful alleles can be found there are also higher, and therefor such PGR are of particular interest. On the other hand, if a crop was successful after domestication and spread rapidly into new areas, local adaptation in combination with human artificial selection may have led to the origin of new, locally adapted varieties outside the center of origin of a crop.
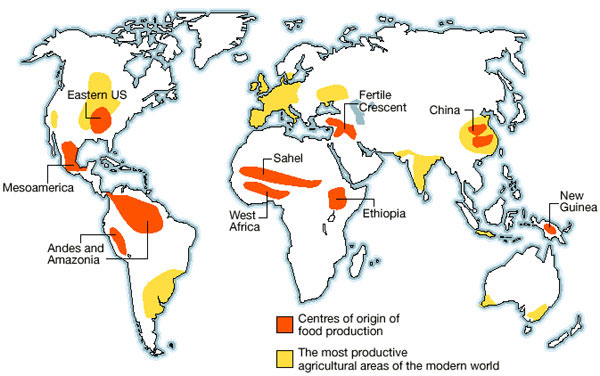
This fact, which applies to many crop species, is a good motivation for allele mining by utilizing the whole diversity present in genetic resources. One example is the improved cold tolerance of a Swiss maize landrace, which originated over the last few hundred years of cultivation in the cool Rhine valley of Switzerland (Peter et al., 2009) and is now evaluated for introgression into modern breeding material (Hölker et al., 2019).
Approaches to allele mining
Several methods are available for allele mining using genetic resources. They are mainly based on the concept of selecting a subset (core collection) of accessions from a large collection of genebank accessions using criteria that either enrich or identify useful genetic variation for a trait or a certain environment. Methods also differ with respect to which type of data are used to investigate subsets of accessions: phenotypic information, genetic information, passport data (geographic coordinates of original sites, ecological information) and climatic data at the original site.
Some approaches are:
- Core collections
- Maximization of genetic, ecological or geographic diversity
- Environmental association mapping
- Identification of association between environmental and phenotypic variation
- Allele mining of known genes
- Utilize geographic distribution or annotation (functional description) and genes and genetic variants to identify novel alleles
- Tests of selection
- Discovery of new genes with a footprint of selection reflecting local adaptation
- Population genetic modeling
- to identify a large number of new alleles at candidate genes
The collection and storage of PGR ex situ is far advanced and reasonably comprehensive even though significant gaps remain (Ramirez-Villegas et al., 2022). Currently, there is a shift towards a characterization and utilization of these resources. Several projects attempt to characterize genetic variation in ex situ genebanks using field trials and analysis of genetic variation. One prominent example is the Seeds of Discovery project at CIMMYT in Mexiko1, which involves the phenotypic analysis of thousands of gene bank accessions of wheat and maize for numerous agronomic traits. An important outcome of these projects are publicly available and digitized resources that can be used by other researchers to identify useful genetic variation in PGR. The underlying accessions can then be obtained from the genebanks and used for further breeding approaches.
In the following some examples and approaches for allele mining that are currently available in the scientific literature are presented.
Query of genebank databases
A very simple approach to increase the chance for identifying useful genetic variation in genetic resources is to query the passport information of gene bank databases. One example is the GENESYS database2 which allows the construction of very specific search queries of publicly available genetic resources. How it works is explained in a short video.3 The ordering information and a KML file4 with the geographic coordinates can be downloaded and used for later analyses.
4 XML-formatted file with geographic coordinates
5 Example taken from http://agro.biodiver.se/2014/07/searching-genesys-the-video/
As an example, one may want to find Aegilops tauschii from Armenia with frost tolerance.5 There are 14 accessions that satisfy those requirements, and they are distributed in the the range shown in Figure 2.
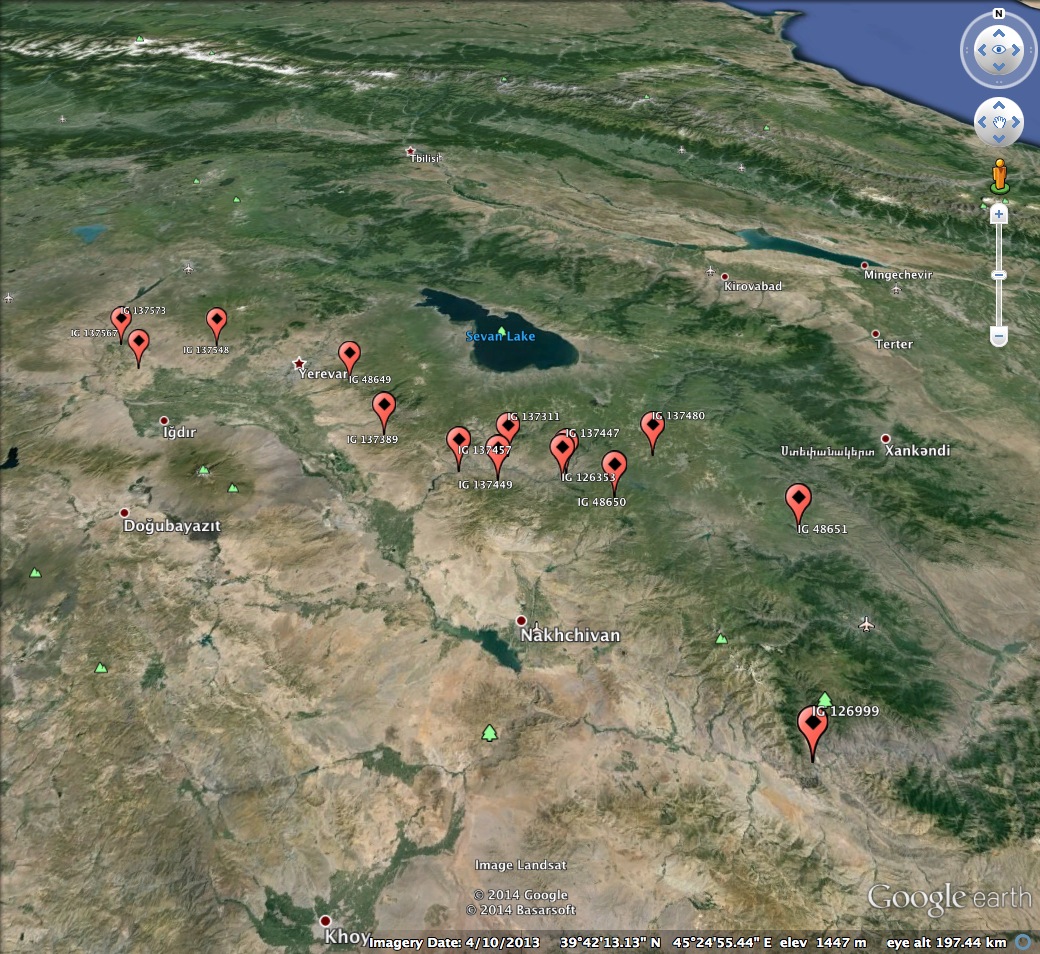
Identification of new disease resistance alleles in wheat
In an application of an allele mining strategy, 733 wheat accessions obtained from the German genebank at IPK Gatersleben, which represent a broad geographic distribution because they originate from 20 countries, were investigated by DNA sequencing and functional assays (classical pathogenicity tests) for new alleles at the Pm3 powdery mildew resistance genes (Bhullar et al., 2010).
Out of 8 cloned sequences of the Pm3 gene from this sample, two new alleles were identified, which can be further tested by backcrossing into elite material. The new alleles were found in accessions from China and Nepal. Figure 3 shows the schematic sequence alignment of previously known and new alleles of the Pm3 gene, of which two were found to be functional in pathogenicity tests.
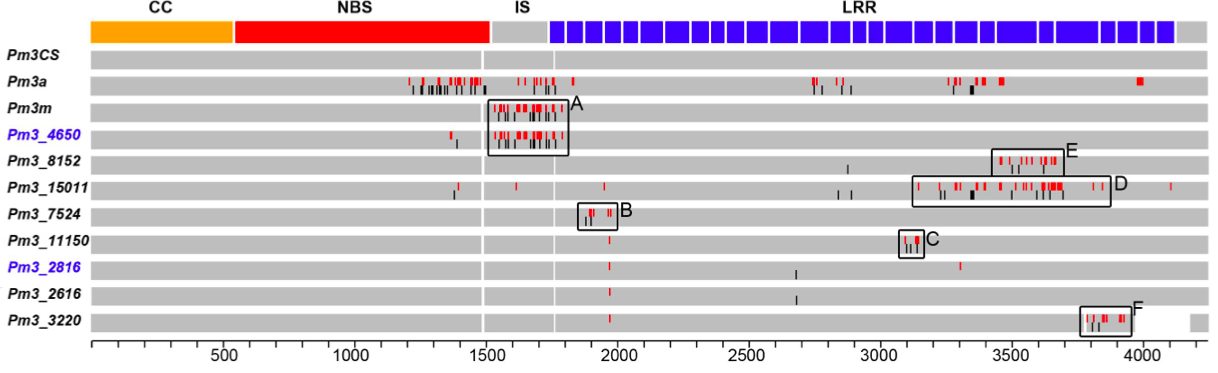
Focused identification of germplasm strategy (FIGS)
A more formalized approach that was shown to work for the identification or new resistance alleles is Focused Identification of Germplasm Strategy (FIGS). This method is based on the hypothesis tha adaptive traits expressed in a genotype (i.e., a genebank accession) reflect the selection pressures of the environment in which it was collected. For this reason, both trait data and environmental data (mostly climate data) are combined to produce an a priori information or hypothesis that reflects a trait-environment relationship. The trait data are obtained from field trials at multiple locations. The key requirement is that the ecogeographic variables act in a similar fashion (i.e., exert the same selection pressure) at each of the source locations of the plant genetic resources studied.
In a second step, a set of accessions is used to define a set of accessions that contain the trait with a high probability. This set is then investigated using more elaborated analyses using a modified version of principal component analysis. Since these additional investigation can be time-consuming and expensive, FIGS is to make allele mining more efficient.
The key outcome of FIGS is a quantitative trait-environment relationship, which is based on the assumption that agro-climate parameters influence (via evolutionary adaptation) adaptive traits that are favorable for agriculture (i.e., time to flowering, drought tolerance).
Examples of FIGS analyses
General structure of a FIGS analysis
In the following one FIGS analysis is described. It is important to note that only the key concept is similar over FIGS studies, but that the data and statistical analyses used may differ between studies. The task in our example was to select a subset of accessions from Nordic (i.e., Scandinavian) barley accessions for further studies.
Based on earlier studies in ecology and plant breeding it was established that ecological niche modeling6 can be used to predict the distribution of wild relatives of crop plants. Other early studies also attempted to correlate phenotypic with climatic variation, but most associations were weak. In the following an outline of a FIGS analysis is shown (Figure 4) as described by Endresen (2010):
6 It describes the range of environmental parameters within which a species can exist. See Wikipedia
- Identify the geographic origin of a large collection of barley landraces from plant genetic resource databases
- Produce field trial data of a selected set of accessions representing a broad and climatically diverse range of regions-of-origin of accessions. Conduct field trials at multiple locations.
- Measure a substantial number of traits (\(>10\)) in the field trials.
- Receive climatic data from climate databases such as the WorldClim database7
- Organise the data into a three-dimensional array with three dimensions: Genotype, trait, as well as year with location.
- Create a second three-dimensional array with the dimensions: Genotype, 12 monthly means of climate data, and a set of climate variables.
- Analyse with the PARAFAC (Generalized PCA for higher order arrays) and N-PLS method (N-way partial least squares for multiway regression).
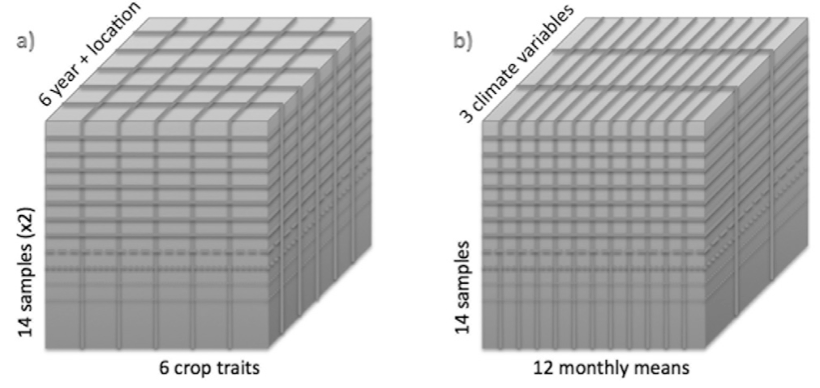
This is a short (advanced) description of the to multivariate analyses previously used for FIGS.
PARAFAC (Parallel Factor Analysis)
PARAFAC is based on a tensor decomposition model, which seeks to approximate a three-way (or higher) data array \(\mathcal{X}_{i,j,k}\) as a sum of \(F\) rank-one components: \[\begin{equation} \label{parafac} \mathcal{X}_{i,j,k} \;\approx\; \sum_{f=1}^F a_{i,f}\,b_{j,f}\,c_{k,f}, \end{equation}\] where \(A=[a_{i,f}]\), \(B=[b_{j,f}]\), and \(C=[c_{k,f}]\) are the factor (loading) matrices for each mode (e.g. germplasm accessions, markers/genes, environments).
The method provides uniqueness without rotation under certain “mild” conditions (e.g. factors being linearly independent), the CP/PARAFAC decomposition is essentially unique, avoiding ambiguous rotations common in matrix PCA. An important characteristic is a multilinear structure, in which each component \(f\) captures a simultaneous pattern across all modes—e.g. a specific allele–environment combination that differentiates a subset of accessions.
The expected output of a PARAFAC analyses are the following:
Factor matrices \(A, B, C\):
- \(A\) scores reveal which accessions carry each latent allele–environment pattern.
- \(B\) loadings show which genes or markers contribute to each pattern.
- \(C\) weights indicate which environmental variables (e.g. climatic descriptors) drive each component.
Core consistency diagnostics: Measures (e.g. CORCONDIA) to select the optimal number of components \(F\).
Explained variation: Percent variance in the original tensor explained by the chosen PARAFAC model.
N-PLS (N-way Partial Least Squares Regression)
N-PLS is an extension by multiway regression and generalisation of partial least square regression (PLS). It models the relationship between an \(N\)-way predictor tensor \(\mathcal{X}\in\mathbb{R}^{I\times J\times K}\) and a response matrix \(Y\in\mathbb{R}^{I\times M}\) (e.g. trait values) via latent (hidden) variables. This is achieved by simultaneous decomposition: At each component \(h\), N-PLS finds weight vectors \(w^{(X)}_h\) (one per mode of \(\mathcal{X}\)) and \(q_h\) for \(Y\) such that the covariance between the projected scores \[\begin{equation} t_h = \mathcal{X} \times_2 w^{(X,2)}_h \times_3 w^{(X,3)}_h,\quad u_h = Y \, q_h \end{equation}\] is maximized. Here, \(\times_n\) denotes the \(n\)-mode tensor–vector product. It also results in *orthogonal deflation** after extracting each component, both \(\mathcal{X}\) and \(Y\) are deflated and remove the effect of \(t_h\) and \(u_h\) to ensure subsequent components capture new covariance.
The expected outputs of N-PLS are
- X-mode weight vectors \(\{w^{(X,n)}\}\): One set per mode, indicating how to project each dimension (accession, marker, environment) onto latent scores.
- Y-weights \(q\): How each response (trait) loads onto latent variables.
- Scores \(T = [t_h]\) and \(U = [u_h]\): Latent representations of accessions in predictor and response spaces, respectively.
- Regression coefficients: A multiway coefficient array \(B\) that can predict \(Y\) from new \(\mathcal{X}\) data.
- Model diagnostics: Cross-validated \(R^2\), RMSEP (root-mean-square error of prediction) per trait, and the number of significant components.
In a FIGS analyses, these methods allow one to:
- PARAFAC: uncover simultaneous “hotspots” of allelic variation and environmental gradients that define subsets of germplasm with putatively adaptive alleles.
- N-PLS: directly link multi-environmental genotypic profiles to measured trait variation (e.g. drought tolerance), facilitating the prediction of promising accessions from the broader collection.
The results of this study showed that for traits such as heading dates, ripening days and harvest index, the missing phenotypes in landraces can be predicted with an acceptable degree of accuracy. However, the number of accessions in the landraces and the extent of field trials used to calibrate the predictive models are very crucial (more trials are better).
Drought-tolerant faba beans
Another study attempted to identify climatic variables that are associated with the drought tolerance in faba beans, Vicia faba Khazaei et al. (2013). Based on genebank passport data (Figure 5), two large sets of differentially adapted faba bean accessions were defined, which were then evaluated for a set of phenotypic traits.
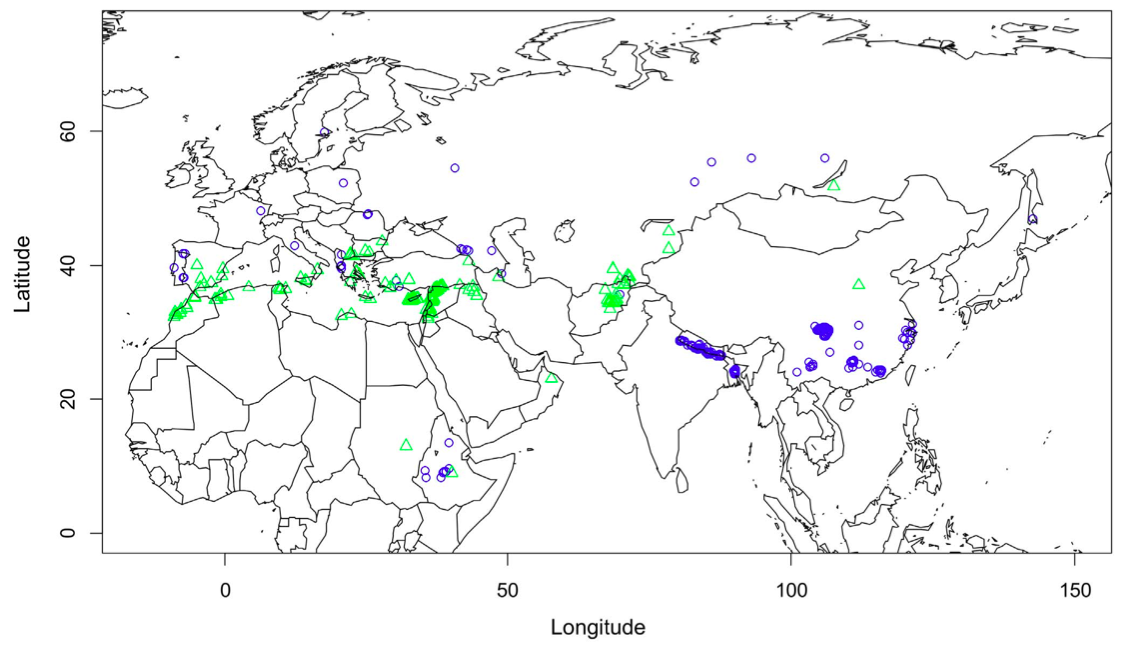
Machine learning algorithms split the accessions into two groups based on phenotypic traits. A comparison of the a priori defined groups based on climatic data from the site of origin and the phenotypic trait distribution indicated a almost perfect match of those groups, which suggested that the climatic information of the site of origin can be used to identify differentially adapted genotypes with a high confidence (Figure 6).
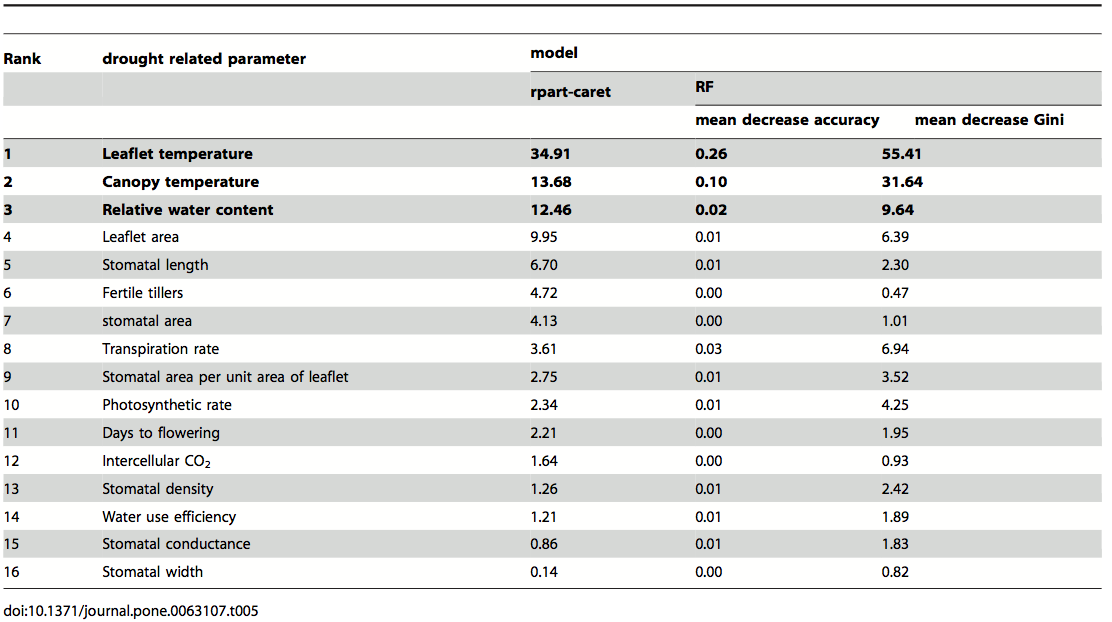
Using FIGS to identify new sources of resistance genes
The study by Bari et al. (2012) investigated the distribution against yellow rust resistance alleles in wheat. Using machine learning approaches such as Support Vector Machines (SVM) to establish a relationship between climate variables and phenotypic data on resistance, a better match than with regression approaches was found. There was a relationship between ecographic variation and the occurrence of yellow rust resistance genes among accessions from different regions. The average success rate of prediction whether a site of geographic origin contained a resistant accession was 76%.
Other examples are the detection of wheat accessions resistant to the Russian wheat aphid (Diuraphis noxia)(El Bouhssini et al., 2011), and stem rust (Puccinia graminis Pers.) in wheat (Triticum aestivum L. and Triticum turgidum L.) and net blotch (Pyrenophora teres Drechs.) in barley (Hordeum vulgare L.) (Filip Endresen et al., 2011). Another study identified new potential sources of resistance genes for the Ug99 stem rust pathogen (Endresen et al., 2012).
Careful reading of these studies, however, indicates that the overall rate of hits is relatively low and that further studies in each crop are required to verify that the observed resistances are real. Furthermore, FIGS is a pure pattern matching approach and does not include information about the genetic history of a crop and the causes of the association between geographic distribution of phenotypes and climatic parameters. For this reason, there is much room for improvement and further research.
Allele mining based on population genetic analysis
Simular to the analysis of geographic and population genetic patterns, the analysis of genetic variation can also be used to identify relevant candidate genes.
One example is the identification of new alleles of a disease resistance gene against barley yellow mosaic virus (Hofinger et al., 2011). The eukaryotic translation initiation factor 4E (eIF4E) gene located on chromosome 3H is a source of resistance to the bymoviruses Barley yellow mosaic virus and Barley mild mosaic virus. Nearly all modern barley cultivars have two recessive eIF4E alleles, rym4 and rym5, that confer isolate-specific resistances.
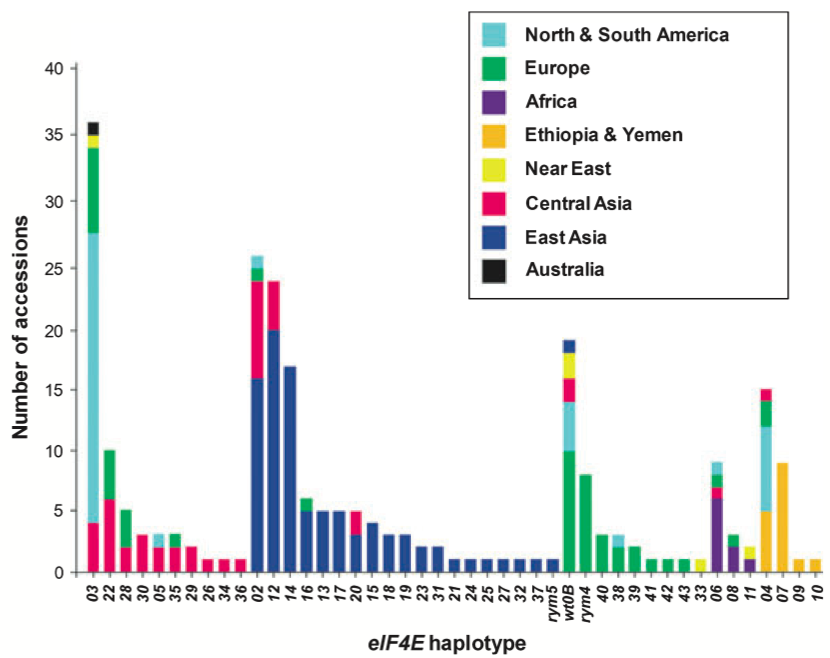
The DNA sequence of eIF4E was analysed in 1,090 barley landraces and noncurrent cultivars originating from 84 countries. A very high nucleotide diversity was found in the coding sequence of eIF4E, and all nucleotide polymorphisms in the coding sequence were amino acid polymorphisms, which is very unusual. A total of 47 different eIF4E haplotypes were identified, and phylogenetic analysis provided evidence of strong positive selection acting on this barley gene.
The eI4FE haplotype diversity was higher in East Asia, whereas SNP genotyping identified a comparatively low degree of genome-wide genetic diversity in 16 of 17 tested accessions (each carrying a different eIF4E haplotype) from this same region. In addition, the calculation of selection statistics using coalescent simulations showed evidence of non-neutral variation for eIF4E in several geographic regions, including East Asia, the region with a long history of the bymovirus-induced yellow mosaic disease.
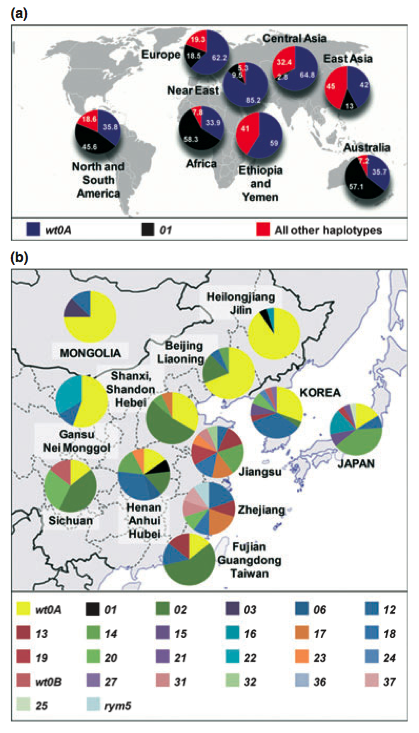
The results indicate that eIF4E may have played a role in local adaptation to different habitats. However, no functional studies of the new resistance alleles were conducted, and for this reason, it was not clear whether the new alleles are really of functional importance or effect.
Key concepts
Summary
- Allele mining is the identification and utilization of useful genetic variation in plant genetic resources.
- The targeted identification of alleles contributes to adapt crops to climate change, allows new regions of cultivation and new uses such as biomass production
- Allele mining is based on the concept that genetic diversity is highest in the center of domestication
- Mining of plant genetic resources includes different types of information: Genotypic and phenotpic data, passport information and climatic data from the site of origin.
- Different methods for the identification of useful genetic variation exist: Core collections, Environmental association mapping to identify novel genes, allele mining of known genes, tests of selection
- The focused identification of germplasm strategy (FIGS) is a multivariate approach for the identification of novel plant genetic resources, that combines data on genetic variation, phenotypic variation and environmental variation.
Further reading
Here are a few further studies on allele mining in plants:
sharwood_mining_2022 - Mining for alleles in photosynthetic traits of plants
Haupt and Schmid (2020) - Example of the combination of FIGS and the generation of core collection
rebetzke_review_2019 - A review on the role of phenotyping in allele mining
wambugu_role_2018 - A review on the role of genomics in allele mining
Study questions
- What are the different approaches for the identification of alleles for the purpose of allele mining?
- Can you identify bottlenecks and limitations in the different approaches for allele mining?
- Why is the resolution and the prediction ability of FIGS limited, and how could it be improved?
- Which traits are more suiof allele mining methods like FIGS or population genetics approaches?
- Why are genetic resources originating from outside the center of domestication of a crop of interest for allele mining?
- Can you think of a further improvement of allele mining approaches with genomics approaches (e.g., genome sequencing or the analysis of gene expression) or functional approaches such as genome editing?
- The eIF4E gene is a highly conserved (i.e., slowly evolving) gene but harbors a large number of amino acid polymorphisms in barley landraces. Can you come up with some explanations for the large number of amino acid polymorphisms? How would you test whether a large number of polymorphisms is unusual or not as evidence that the polymorphisms reflect different resistance alleles agains the barley yellow mosaic virus?